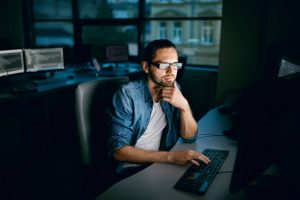
In an earlier post, we looked at some definitions of reference data and focused on a description of how reference data sets are used. We also considered the relevant characteristics you can use to determine if a data set could be designated a reference data set. Those specifications for reference data are satisfactory for strict operational purposes (such as segregating reference data sets and managing them separately as corporate shared resources). But three recent developments are likely to influence not only how organizations manage reference data, but how the concept of reference data may redefine operational data governance altogether. Those three developments are:
- Data lakes.
- Democratized analytics.
- The growing need for data protection.
Data lakes
Let’s start with data lakes. Fundamentally, a data lake is intended to be a repository for data objects in their native format. The idea is to make the data available to different consumers who want to consume it in ways specific to their needs. Practically, without any type of oversight, a data lake is more likely to degenerate into a “data dump” that holds numerous data assets with no rhyme or reason due to its (lack of) organization. More on this in a minute.
Democratized analytics
Next: the concept of democratization of analytics. Twenty years ago, only a few people could use advanced algorithms for predictive analytics. Those were the people who created the algorithms, or the people who invested enough time to understand and then code the algorithms into workable applications. Today, the plethora of user-friendly predictive analytics engines and products has lowered the barrier of entry to advanced analytics. The only barriers now are the massive volumes of data and the broad variety of content the data assets contain.
Data protection
Finally, there is a heightened awareness about the need to manage and protect sensitive data. You may be focused on regulatory compliance – such as that required by the EU’s General Data Protection Regulation (GDPR), or by newer laws such as the California Consumer Privacy Act (CCPA). You probably recognize how indignant consumers have become about ungoverned corporate attempts to monetize their personal data. And you're most likely concerned about the rising number of high-volume data breaches. Whatever the case, there is growing urgency to improve ways of complying with information protection policies.
The link with reference data
OK, so what does all this have to do with reference data? Ultimately, everything. Each of these developments is limited by the scale of data volume and variety. Ungoverned data lakes are essentially useless unless there is some ontology imposed to help organize the information by topic. Analytics is not truly democratized unless all data consumers can rapidly find the data assets they need to perform their analyses or configure their reports. And how can you assert compliance with data protection laws unless you know which data assets contain sensitive data?
Organizing data assets with reference data
We can use reference data as the basis for data asset organization. Each conceptual reference domain is represented by its collection of values in the corresponding value domain. So, by scanning a data asset for reference values, it's possible to make automatic inferences about content topics.
For example, consider CCPA’s definition of personal data that includes residential address. Geographic values (such as states, cities and street address names) are all parts of defined reference data sets. Scanning a data asset and finding many matches to these reference domains implies there are addresses in the data asset with potential data sensitivity. Alternatively, assessing structured data assets and aligning columns by reference domain can help you create a topic index. In turn, data consumers looking for particular bits of information for their reports and analyses can search the index to find what they need. And these topics can be folded into an operational ontology for organizing the data in a data lake.
In essence, reference data is much more than a defined set of permissible values. Reference data could be the foundation of future governance structures. Adopting reference-data-based ontologies for data organization and inferences also raises data literacy, reduces data organization complexity and simplifies regulatory compliance.
Learn more about SAS solutions. Download The SAS Data Governance Framework