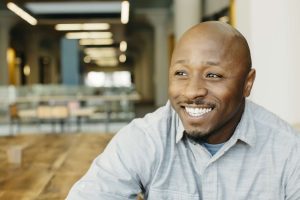
In my last post I talked about how to make data quality dimensions work for you. I focused on the accuracy dimension because it’s a topic that draws a lot of heated debate amongst practitioners. So I wanted to outline a pragmatic way forward for organisations starting out with their data quality definitions. This week I wanted to share a very simple technique you can use to proactively improve data accuracy. As a recap, I consider data accuracy as the measure of whether a piece of information reflects reality (or an authoritative, trusted source).
Data quality tools can perform many functions, but obviously they can’t physically get in a car and check whether Nancy Roberts still lives at 3 Elm Crescent, Chiswick. Data profiling can tell you whether a serial number has been formatted incorrectly, but it can’t tell you whether it is the correct serial number for the power unit situated on the third floor next to the fire hydrant or whether there is even a power unit located there at all!
Where data quality tools can help to improve data accuracy, of course, is the discovery and management of data quality rules. That’s what we’re really doing with data profiling – discovering rules. Once we document and agree on these rules, we can then start to assess the quality of our data and take action when we find anomalies. Data profiling finds the rules and data quality assessment measures the performance against our rules.
Of course, we can have perfectly valid data that lacks accuracy. Our serial number could be formatted beautifully, but the power unit could have been swapped out 12 months earlier and the records never updated. This is why my past site accuracy surveys often found huge issues with data quality accuracy. I’ve witnessed figures as high as 40 percent inaccurate data, and of course all this inaccuracy costs the organisation in wasted operating costs, increased capital expenditure, reduced customer service levels and an overall hit on profits.
So how can you improve accuracy?
A simple technique that you can adopt is to increase the frequency and quality of reality checks. The technique works on the basis that every item of data will experience data quality degradation if there are no reality checks at some point in the future.
For example, on Data Quality Pro we routinely discover inaccurate emails from our members who have changed employer but not updated their email. When this happens we try to reach out to them and see if they want to improve their own data accuracy by updating their email, but of course this can be a costly and laborious process. It’s a reactive process, so we’re looking at ways to make it easier for members to update their own details. But of course a balance has to be made; it’s easy to annoy people if you remind them too often.
If we wanted to perform reality checks on equipment, these site visits can soon become expensive. But there are alternatives. One tactic I’ve seen several case studies for is the use of "BAU-reality-checks" when performing site maintenance, for example.
The idea is a simple one: If you’re working on a "business as usual" task, such as repairing or installing a piece of equipment, check that its information and that of the surrounding equipment is accurate. If you have data quality tools, use them to add "hotspot" markers to surrounding data to flag suspect values such as poorly formatted or incomplete data.
For example, if a field engineer is topping up battery acid on a power supply, he often has a few minutes to spare at the end of the field work to quickly validate all the data relating to the equipment he has just serviced. This is far more cost-effective than performing ad hoc site visits just for data quality work alone. If your data quality tools have highlighted equipment with a higher probability of defective data, then they can also prioritise that information for a reality check, too.
You can adapt your reports and data distribution processes so that people can verify the accuracy of data when carrying out any number of data-driven tasks such as:
- Receiving queries from customers in call centres.
- Delivering supplies to retailers.
- Messaging customers when they log into their account details online.
The idea is that by increasing both the frequency and quality of your reality checks, it’s less likely for your data to become inaccurate. You can never entirely prevent inaccuracy, but the scale of the problem is significant (and largely hidden) in most organisations. So with a little creativity, hopefully you can gain visibility and greater control of the problems around improving data accuracy.
How are you increasing the frequency and quality of your reality checks? Welcome your views.
Read another post on data accuracy
1 Comment
The data accuracy causing big problem for businesses and the blog is write this one good way to lessen it. However, I have long been data driven person and believe in its values while its accurate at same time work up such data that can be available and it more accurate for a use in business making decision.
Having said that, and I am not referring only to equipment data but to data in general. Here, I believe are initiative that solve some data accuracy problems.
1. Know the data you are working with so as you can develop the necessary model for programming
2. Apply the technology that are useful at this matter (Big Data)
3. Math...Math and Math is the focus...
4. While implementing the solution develop a process that is phases and use business categories
and references for further data accuracy development.
These are some highlight in developing which shall provide me a reach and goals in generating corrected data if you are interested let us discuss them.
Best Regards.