In this 5-part blog series on the Big Data Cheat Sheet on Hadoop, we’re taking a look at these five questions from the perspective of a marketer:
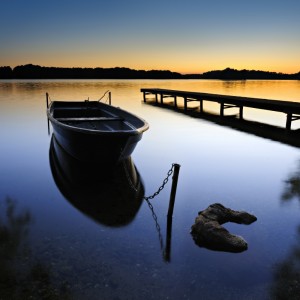
- What can Hadoop do that my data warehouse can’t?
- Why do we need Hadoop if we’re not doing big data?
- Is Hadoop enterprise-ready?
- Isn’t a data lake just the data warehouse revisited?
- What are some of the pros and cons of a data lake?
We’ve already tackled the first three questions, and we’re now on question 4, so it’s time to talk about the data lake.
Question 4: Isn’t a data lake just the data warehouse revisited?
Some of us have been hearing more about the data lake, especially during the last six months. There are those that tell us the data lake is just a reincarnation of the data warehouse—in the spirit of “been there, done that.” Others have focused on how much better this “shiny, new” data lake is, while others are standing on the shoreline screaming, “Don’t go in! It’s not a lake—it’s a swamp!”
All kidding aside, the commonality I see between the two is that they are both data storage repositories. That’s it. But I’m getting ahead of myself. Let’s first define data lake to make sure we’re all on the same page. James Dixon, the founder and CTO of Pentaho, has been credited with coming up with the term. This is how he describes a data lake:
“If you think of a datamart as a store of bottled water – cleansed and packaged and structured for easy consumption – the data lake is a large body of water in a more natural state. The contents of the data lake stream in from a source to fill the lake, and various users of the lake can come to examine, dive in, or take samples.”
And earlier this year, my colleague, Anne Buff, and I participated in an online debate about the data lake. My rally cry was #GOdatalakeGO, while Anne insisted on #NOdatalakeNO. Here’s the definition we used during our debate:
“A data lake is a storage repository that holds a vast amount of raw data in its native format, including structured, semi-structured, and unstructured data. The data structure and requirements are not defined until the data is needed.”
The table below helps flesh out this definition. It also highlights a few of the key differences between a data warehouse and a data lake. This is, by no means, an exhaustive list, but it does get us past this “been there, done that” mentality:
Let’s briefly take a look at each one:
- Data. A data warehouse only stores data that has been modeled/structured, while a data lake is no respecter of data. It stores it all—structured, semi-structured, and unstructured. [See my big data is not new The data warehouse can only store the orange data, while the data lake can store all the orange and blue data.]
- Processing. Before we can load data into a data warehouse, we first need to give it some shape and structure—i.e., we need to model it. That’s called schema-on-write. With a data lake, you just load in the raw data, as-is, and then when you’re ready to use the data, that’s when you give it shape and structure. That’s called schema-on-read. Two very different approaches.
- Storage. One of the primary features of big data technologies like Hadoop is that the cost of storing data is relatively low as compared to the data warehouse. There are two key reasons for this: First, Hadoop is open source software, so the licensing and community support is free. And second, Hadoop is designed to be installed on low-cost commodity hardware.
- Agility. A data warehouse is a highly-structured repository, by definition. It’s not technically hard to change the structure, but it can be very time-consuming given all the business processes that are tied to it. A data lake, on the other hand, lacks the structure of a data warehouse—which gives developers and data scientists the ability to easily configure and reconfigure their models, queries, and apps on-the-fly.
- Security. Data warehouse technologies have been around for decades, while big data technologies (the underpinnings of a data lake) are relatively new. Thus, the ability to secure data in a data warehouse is much more mature than securing data in a data lake. It should be noted, however, that there’s a significant effort being placed on security right now in the big data industry. It’s not a question of if, but when.
- Users. For a long time, the rally cry has been BI and analytics for everyone! We’ve built the data warehouse and invited “everyone” to come, but have they come? On average, 20-25% of them have. Is it the same cry for the data lake? Will we build the data lake and invite everyone to come? Not if you’re smart. Trust me, a data lake, at this point in its maturity, is best suited for the data scientists.
Why this matters
At the most fundamental level, big data is mostly driven by customer-related activity, and Hadoop is a very effective way to handle big data. And as a marketer, you may hear rumblings that your organization is setting up a data lake and/or your marketing data warehouse is a candidate to be migrated to this data lake. It’s important to recognize that while both the data warehouse and data lake are storage repositories, the data lake is not Data Warehouse 2.0 nor is it a replacement for the data warehouse.
So to answer the question—Isn’t a data lake just the data warehouse revisited?—my take is no. A data lake is not a data warehouse. They are both optimized for different purposes, and the goal is to use each one for what they were designed to do. Or in other words, use the best tool for the job.
This is not a new lesson. We’ve learned this one before. Now let’s do it.
This is the 4th post in a 5-part series, "Big Data Cheat Sheet on Hadoop." This spin-off series for marketers was inspired by a popular big data presentation I delivered to executives and senior management at a recent SAS Global Forum Executive Conference.
Editor’s note:
If you did not read the previous posts in this series, I encourage you to read those as well. Tamara's goal with this series is to enable you to have an informed view of how this area of technology can support your strategy. Armed with these perspectives, hopefully you can partner even more closely with I.T. and operations to deliver the best possible customer experience.
As Tamara puts it, Hadoop is here to stay and it’s ready to “play” with your enterprise data warehouse. Download her Non-Geek’s Big Data Playbook to figure out which use cases make sense for your organization. She wrote the playbook for the technologically-savvy business professional who prefers pictures to words, simplicity to complexity, and briefer explanations to longer ones. Once you're comfortable with Hadoop and want to delve deeper into analytically-driven marketing solutions, start with our Customer Intelligence home page at: www.sas.com/customerjourney.
And as always, thank you for following!
5 Comments
"relational data warehouse stores data in a hierarchical manner". Really? It's quite an interesting statement, since RDBMS have no specific mention to a "hierarchical manner" (whatever it could mean) to store data. Physical Data Independence (https://en.wikipedia.org/wiki/Codd%27s_12_rules rule 8) was defined exactly to avoid to tie RDBMS to physical specification that surely change as time goes by. The only "hierarchy" that I can think of is a B-Tree. But that's a tree, not a hierarchy. And B-Trees are also available in Hadoop (http://wiki.apache.org/hadoop/HadoopOverview).
So now, the result is that this article, instead of helping people to understand, simply generates even more confusion, and, even worse, contribute to spread wrong ideas, leading people to wrong decision. I would expect from SAS, a company that I highly respect, more scientific and correct contributions, not just marketing bloatware.Thanks.
Thank you, Davide, for your feedback. I understand your point and can see how it might be confusing for more technically-savvy readers who are familiar with data storage methods. I’ve updated the post to highlight a better and more relevant storage comparison for this blog’s non-technical audience.
Pingback: Marketers ask: What are some of the pros and cons of a data lake? - Customer Analytics
Pingback: Marketers Ask: What Are Some of the Pros and Cons of a Data Lake? | The Cyberista Says
Pingback: Webinars su Data Lake e U-SQL – UGISS