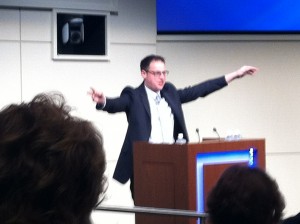
I consider myself lucky to have confirmed first-hand that Nate Silver is a relatively smart guy.
He most recently published a book, The Signal and the Noise: Why So Many Predictions Fail – but Some Don’t, and he is particularly well known for accurately predicting the outcome of the 2012 U.S. Presidential election 51 times in parallel (all 50 states and the District of Columbia).
His work is not limited to politics, also having established views on sports and economics. And what I found remarkable is how he structures his views on big data, as well as the way he demonstrates the importance of relativity in the thoughtful analysis of data and applying its findings.
Historical Relativity
Nate calls attention to the historical precedent to what we’re currently experiencing with big data – specifically the era that saw the invention of the printing press. Not surprisingly, that invention in Europe ushered in a flourishing in literacy at a time and place that was also experiencing the Renaissance, the Protestant Reformation and the Age of Exploration. In the same time period, Europe also saw the bloodiest century in history, with epic political struggles and wars among the French, English, Ottomans, the Spanish-led Holy Roman Empire and the Catholic Church. That original era of “big data” didn’t produce “big progress” at first, and instead produced a lot of conflict as people were trying to sort out this whole new wealth of information.
His analogy is certainly interesting because in today’s world, there is a sense that much of the promise of big data is yet to be realized, while it’s fairly well established that high performance analytics and the expansion of affordable computing power and data storage capacity are the keys to unlocking big data’s value. At the same time, the battle lines are being drawn and sparks are flying among the "Tech Titans" (Amazon, Apple, eBay , Facebook and Google) as they jostle for commercial dominance of the consumer economy.
Scientific Relativity
From Nate’s perspective, he is skeptical about what happens when you have a lot of data but not a lot of people who know what to do with it. One risk you run is to have a lot of “false positives” - in those cases the models might explain something but are not useful for accurate prediction. To illustrate, he cites an article by John P.A. Ionnidis, Why Most Published Research Findings are False, in which the author used Bayesian statistics to predict that most findings in academic journals could not be replicated or verified and therefore could not make good predictions.
Bayer Laboratories apparently did a test of that hypothesis on results that were deemed to be statistically significant in medical journals, and they found that they could not replicate two thirds of the experiments. From that, Nate draws the conclusion that data mining could be a good thing, but it also means that you can sometimes mistake a noisy, fortunate, “lucky relationship” for a real signal. Even Google has had some problems in over-predicting flu outbreaks this past year based on their algorithms, potentially placing too much reliance on certain search terms to predict outbreaks. That flaw became apparent once CDC data became available, showing that at a certain point there was a drop-off in flu cases, where the Google-driven prediction showed further spread.
Political Relativity
It’s clear that part of Nate’s “secret sauce” in terms of political predictions relates to how he handles bias in polls and in the reporting of poll outcomes. One clue came from how he zeroed in on the 2012 election swing states and displayed the predictability of partisan polling by state - not surprisingly showing that republican-biased polls predicted mostly Romney wins and democratic-biased polls predicted mostly Obama wins. This is significant in our current environment where people have gravitated toward news sources where the reporting resonates with their views, highlighting the correlation between the partisan atmosphere in Congress and the emergence of partisan-biased news media – notably Fox News and MSNBC.
And then there’s the presidential election and the vagaries of the Sunshine State, which will long be remembered for its role in holding up the very close year 2000 presidential election between Al Gore and George W. Bush. In the 2012 election, Florida was again one of the “swing states” that both major party candidates deemed as “must win.” Nate somewhat humbly reveals that his prediction of Obama winning Florida was largely a judgment call he made (that made him “look really smart”) because his poll data showed only a 50.1% chance of winning that state - essentially a “coin flip.” He briefly claims bragging rights by revealing that “Nate Silver” beat “Joe Biden” as a searched-for term after his appearance on “The Daily Show.” He was quick to add that both terms are relatively insignificant when compared to “Justin Bieber,” indicating the relative mind-share of politics versus pop culture even in an election year.
The Relative Complexity of Signals and Noise
Problems with data include the sheer complexity that comes with adding variables to any model because as you increase the number of data points, you have an exponential increase in the number of relationships to look at.
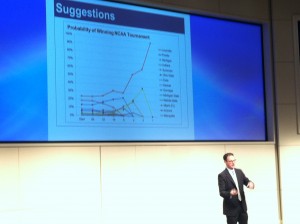
In a very simplistic model, if you are testing for relationships between any 5 variables, there are 10 two-way tests to run, shown in the equation (5x4)/2 = 10. If you double the number of variables to 10, you more than quadruple the number of relationships to test, shown by (10x9)/2 = 45. With that thought in mind, consider the complexity of the Federal Reserve website that tracks 61,000 statistics in real time, creating a potential 1.86 Billion relationships to analyze. That's big data!
The problem is that many of those relationships may be redundant or trivial, and hidden among them are the “real nuggets,” or "signals." It turns out that the “true signals” are not increasing as fast as the number of tests that you can run, and so a gap has emerged between what we think we know and what we really know.
Even when facing big data, we do have the ability to detect patterns, but we can run into trouble by not accepting the limitations of our ability to filter out noise. As a result, we need to be careful not to over-fit a model and lose the ability to predict by being over-focused on connecting all the data points because some of them are truly noise. Faced with this situation, we might be tempted to turn it all over to computers, but that’s not the answer either because we’d forgo peoples’ ability to make inferences. Where machines can be work-saving devices, they cannot replicate our ability to see the bigger picture.
3 Factors Needed to Manage Relatively Big Data:
Going back to the historical precedent of big data, it took about 300 years for the full impact of the printing press to be felt in society. While the similar phenomenon in today’s big data environment will most assuredly take less than 300 years, three factors emerge as necessary catalysts to enable the benefits of big data: context, culture and competition. Per Nate, industries that are successful at using big data that are making profits tend to get all 3 of these elements, and not just one or two of them.
- Context is the accumulation of knowledge enabled by computing power and high performance analytics. In the middle ages, it was the printing press that enabled the first creation of a history of data that provided strong context, ushering in the Bayesian idea of weighing new information against what we already know. Remove the context and with all the information being very new, you get lots of false starts and failed models. Without a context to calibrate to, a culture of analytics and the unbridled competition without good contextual skills can lead to low productivity.
- Culture is an environment that encourages the pursuit of science and insight, which came about initially in the Renaissance – a movement already under way when the printing press was invented. Remove culture, and “death by internal politics” results because you lack the factor that insists on respect for the analytics. Want ideas on how to implement a culture of analytics? Check out this whitepaper.
- Competition is the emergence of a market economy where people can test their ideas and have different incentives to bring their ideas to market. The great wars and strife present in Europe in the middle ages created an element of competition that spurred creativity. If you remove competition, you can get ivory tower sluggishness from having just culture and context. In this respect, it’s very important to test our ideas by making predictions.
In all cases, the relationship between the three factors is not additive, but multiplicative so the potential benefits are far greater than what they might initially seem. You need to have all 3 in order to find the signal among the noise, which is a big part of the explanation why so many predictions fail, but some don’t.
Seeking to encourage all 3 factors in the enterprise is a good way to capitalize on the promise of big data. Other suggestions for how to manage big data include:
- Retain a Broad Perspective
As you consider your data strategy, take steps to retain a broad perspective because nothing in business happens in a vacuum so neither should your analysis. For example, Nate suggests applying Bayes theorem in how you weigh new information against what you already know. Practically speaking, that could be a matter of using your acumen to understand when you’ve reached diminishing returns in your model and making a decision to complete the picture with your own experience. - Encourage Trial and Error
There is a healthy amount of risk-taking you can encourage in the enterprise. Nate highlights the way Google uses A/B testing frequently to resolve disputes between two internal points of view. It can be productive to foster an environment where there’s willingness to try and err, but you need to balance that with knowing that mistakes get more costly as complexity increases. There comes a time where getting more accuracy can take more effort than it’s worth and you can make great progress by eliminating 20% of your biggest mistakes. - Be Aware of Biases
Even your own biases can be a source of problems because any form of bias can take your focus off what really matters. An important way to do that is to be equally aware of where you’re not looking as you are of where you’re are looking. Don't be afraid to ask probing questions - even of yourself.
Nate Silver was the keynote speaker at Prosper – the 2013 SAS Financial Services Executive Summit, and he will also be the keynote speaker at the upcoming 2013 SAS Government Leadership Summit in Washington, DC. As always, thank you for following and let me know what you think!
2 Comments
Just wanted to say this is one of the most thoughtful and well written pieces on a big data approach I have read and I've read a pretty big number (one of our clients is a data agency). Brings to mind the old saying 'we don't see the world as it is, we see it as we are'. Thanks for this excellent read !
Thanks, Chris. I appreciate your kind words and thank you for following!