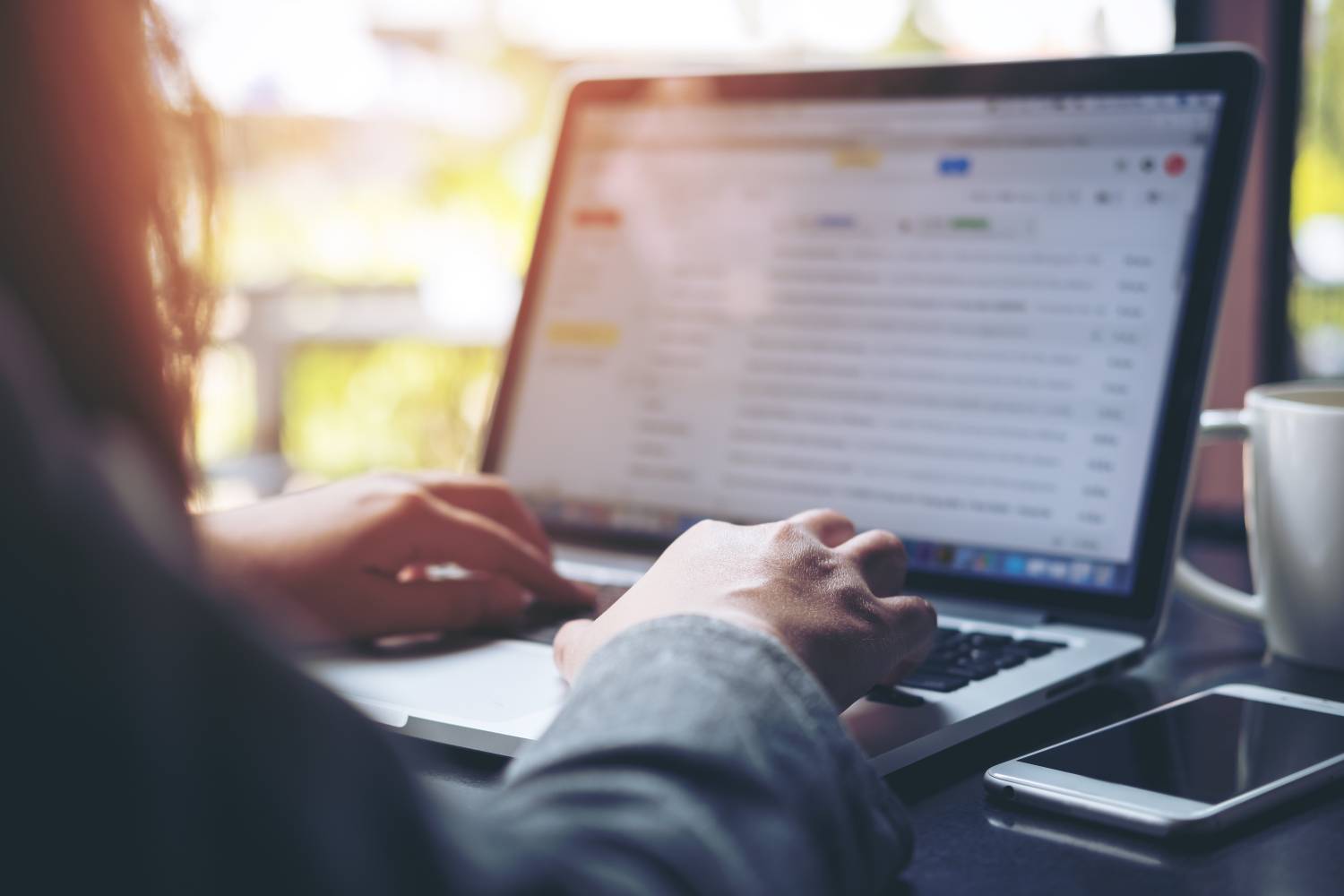
Expect to lose time if you don't include a data steward in your project until you're reviewing the data model.
Expect to lose time if you don't include a data steward in your project until you're reviewing the data model.
Joyce Norris-Montanari discusses data engineers in the second of her two-part series.
Data scientists need good skills in communication, data mining, data wrangling and more. Joyce Norris-Montanari explains.
Joyce Norris-Montanari cites five things she considers essential to data management for analytics.
Focus on data governance, quality and storage if you want to do data management for analytics right.
Before grabbing that streaming data so quickly, Joyce Norris-Montanari says you should ask a few questions.
Joyce Norris-Montanari shares tips to help those who are still working to address GDPR requirements.
Joyce Norris-Montanari talks about how to implement data privacy policies, procedures and guidelines.
Joyce Norris-Montanari shares insights and tips about data security and privacy.
Joyce Norris-Montanari lists personal data considerations related to GDPR compliance.
Joyce Norris-Montanari starts this two-part series with some suggested actions you can take to help you comply with the GDPR.
Four things clients say could go wrong when you're working to become a data-driven business.
Joyce Norris-Montanari defines data-driven design and asks if it's more about technology, processes or mindset.
Joyce Norris-Montanari says IT and business need to work together when giving business users self-service data preparation tools.
To do their jobs well, Joyce Norris-Montanari says users doing analytical data preparation need access to both standardized and raw data.
Managing big data doesn't always mean hiring more people and buying new tools.
Joyce Norris-Montanari says focus on data quality and governance, privacy and security when providing data on demand.
Joyce Norris-Montanari explains why it's so important to pick the right tools to manage your big data.
Joyce Norris-Montanari poses the question: Is Hadoop/big data technology actually ready for MDM?
To show how they're compliant with regulatory mandates, organizations first need an enterprise data strategy. Joyce Norris-Montanari discusses the issues.
In the previous three blogs in this series, we talked about what metadata can be available from source systems, transformation and movement, and operational usage. For this final blog in the series, I want to discuss the analytical usage of metadata. Let’s set up the scenario. Let's imagine I'm a
As I discussed in the first two blogs of this series, metadata is useful in a variety of ways. Its importance starts at the source system, and continues through the data movement and transformation processes and into operations. Operational metadata, in particular, gives us information about the execution and completion
In the first blog of this four-part series, we discussed traditional data management and how we can apply these principles to our big data platforms. We also discussed how metadata can help bridge the gap of understanding the data as we move to newer technologies. Part 2 will focus on
Traditional data management includes all the disciplines required to manage data resources. More specifically, data management usually includes: Architectures that encompass data, process and infrastructure. Policies and governance surrounding data privacy, data quality and data usage. Procedures that manage a data life cycle from creation of the data to sunset
In Part 1 of this series, we defined data governance as a framework – something an organization can implement in small pieces. Data management encompasses the disciplines included in the data governance framework. They include the following: Data quality and data profiling. Metadata (business, technical and operational). Data security. Data movement within the enterprise.
Lately, the definitions of data governance and data management look very much alike. In this two-part series, we'll define data governance and data management. And we'll see that there's a big difference in the two.
In Part 1 of this two-part series, I defined data preparation and data wrangling, then raised some questions about requirements gathering in a governed environment (i.e., ODS and/or data warehouse). Now – all of us very-managed people are looking at the horizon, and we see the data lake. How do
I'm a very fortunate woman. I have the privilege of working with some of the brightest people in the industry. But when it comes to data, everyone takes sides. Do you “govern” the use of all data, or do you let the analysts do what they want with the data to
In my last post, I talked about how data still needs to be cleaned up – and data strategy still needs to be re-evaluated – as we start to work with nontraditional databases and other new technologies. There are lots of ways to use these new platforms (like Hadoop). For example, many
If your enterprise is working with Hadoop, MongoDB or other nontraditional databases, then you need to evaluate your data strategy. A data strategy must adapt to current data trends based on business requirements. So am I still the clean-up woman? The answer is YES! I still work on the quality of the data.