One area that often gets overlooked when building out a new data analytics solution is the importance of ensuring accurate and robust data definitions. This is one of those issues that is difficult to detect because unlike a data quality defect, there are no alarms or reports to indicate a
Author
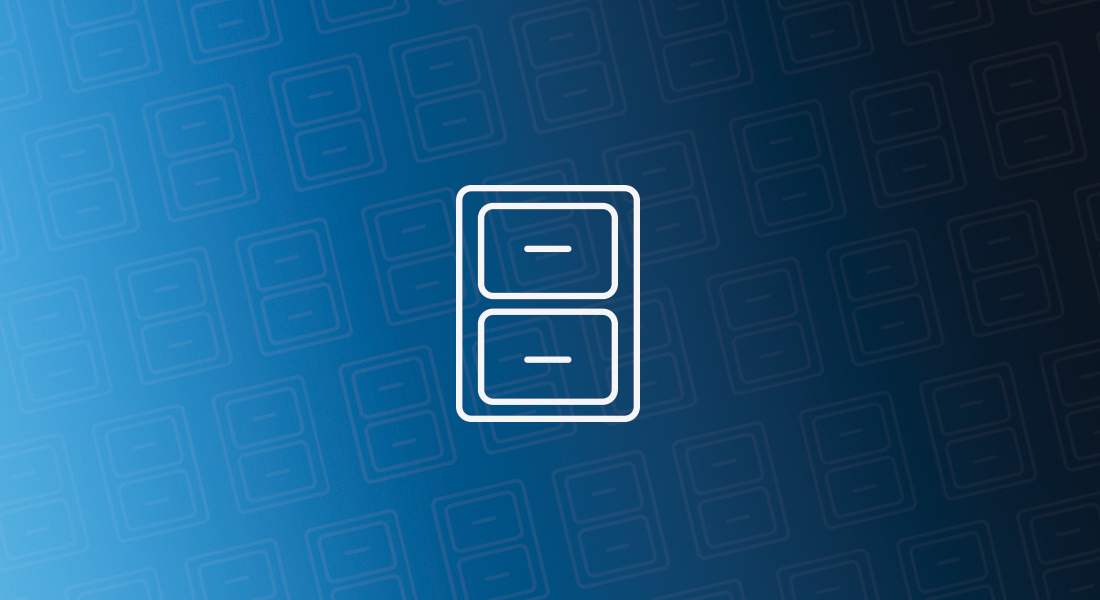
The adoption of data analytics in organisations is widespread these days. Due to the lower costs of ownership and increased ease of deployment, there are realistically no barriers for any organisation wishing to exploit more from their data. This of course presents a challenge because the rate of data analytics adoption
One thing that always puzzled me when starting out with data quality management was just how difficult it was to obtain management buy-in. I've spoken before on this blog of the times I've witnessed considerable financial losses attributed to poor quality met with a shrug of management shoulders in terms
One of the common traps I see data quality analysts falling into is measuring data quality in a uniform way across the entire data landscape. For example, you may have a transactional dataset that has hundreds of records with missing values or badly entered formats. In contrast, you may have
Regulatory compliance is a principal driver for data quality and data governance initiatives in many organisations right now, particularly in the banking sector. It is interesting to observe how many financial institutions immediately demand longer timeframes to help get their 'house in order' in preparation for each directive. To the
One of the significant problems data quality leaders face is changing people's perception of data quality. For example, one common misconception is that data quality represents just another data processing activity. If you have a data warehouse, you will almost certainly have some form of data processing in the form
It’s common at the start of a new year to create a long list of resolutions that we hope to achieve. The reality, of course, is by February those resolutions will likely be a distant memory. The key to making any resolution stick is to start small. Create one small
We've explored data provenance and the importance of data lineage before on the Data Roundtable (see here). If you are working in a regulated sector such as banking, insurance or healthcare, it is especially important right now and one of the essential elements of data quality that they look for
Here on the Data Roundtable we've discussed many topics such as root-cause analysis, continual improvement and defect prevention. Every organization must focus on these disciplines to create long-term value from data quality improvement instead of some fleeting benefit. Nowhere is this more important than the need for an appropriate education strategy, both in
When you examine where most data quality defects arise from, you soon realise that your source applications are a prime culprit. You can argue that the sales team always enter incomplete address details, or the surgeons can't remember the correct patient type codes but in my experience the majority of
Many managers still perceive data quality projects to be a technical endeavour. Data being the domain of IT and therefore an initiative that can be mapped out on a traditional project plan with well-defined exit criteria and a clear statement of requirements. I used to believe this myth too. Coming
Data migrations are never the most attractive of projects to sponsor. For those who have sponsored them previously, migrations can be seen as a poison chalice. As for the first-timers, data migration initiatives are often perceived as a fairly insignificant part in a far grander production. The challenge with data
A lot of data quality projects kick off in the quest for root-cause discovery. Sometimes they’ll get lucky and find a coding error or some data entry ‘finger flubs’ that are the culprit. Of course, data quality tools can help a great deal in speeding up this process by automating
One of the big problems with data migration projects is that, to the outside sponsor, they appear very much like a black box. You may be told that lots of activities and hustle are taking place, but there isn’t a great deal to show for it until the final make-or-break
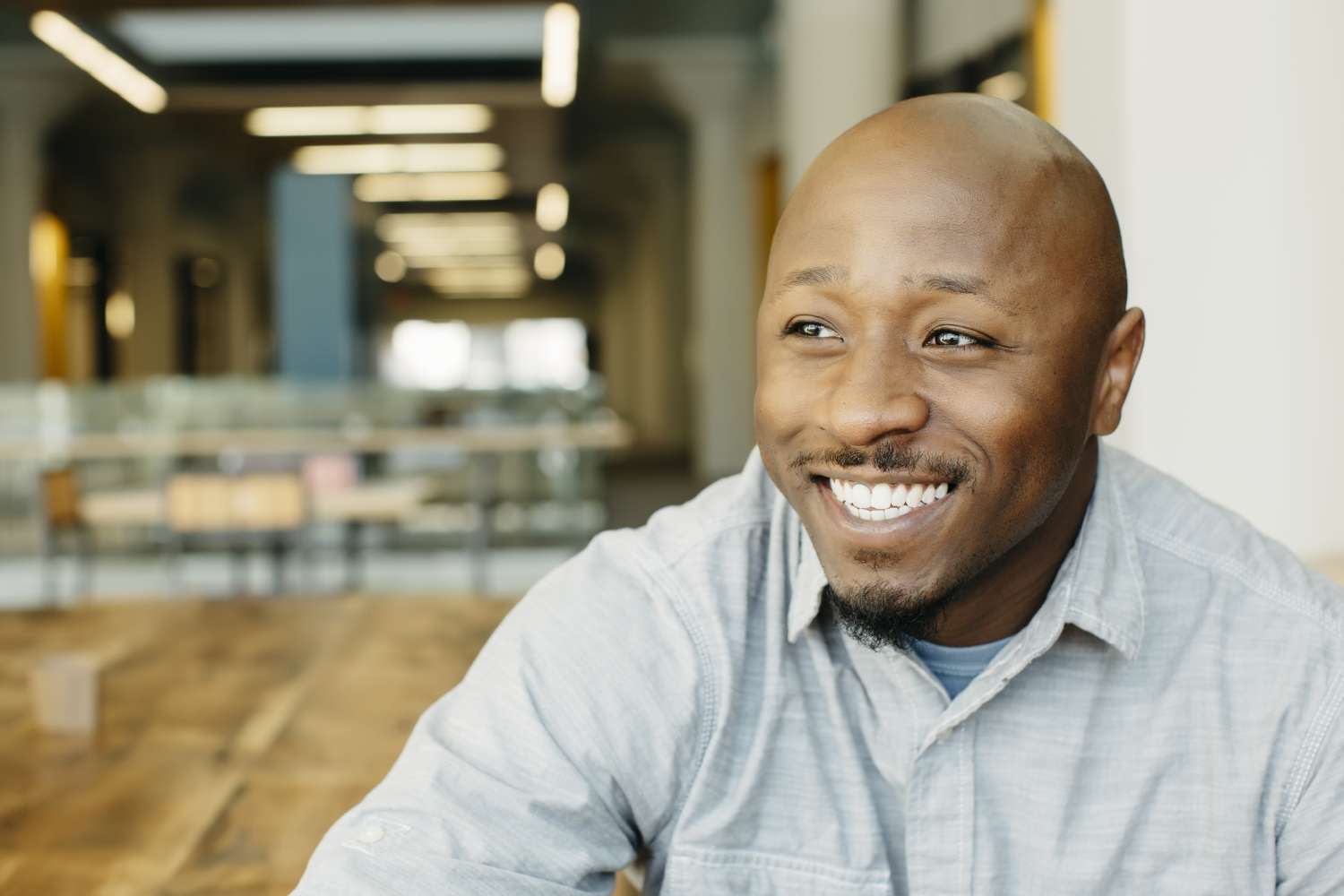
Dylan Jones says one way to improve data accuracy is to increase the frequency and quality of reality checks.
One of the benefits of running an online data quality and data governance community is that over the course of many interviews, you start to see common threads and patterns emerging in the way practitioners create success in their data-driven programs. Data governance is a relatively new discipline, so it’s
In my last post I discussed one of the important traits that I feel truly great data stewards possess – the ability to effect change. Today I want to talk about how you actually identify, train and nurture everyday workers into the role of data stewards. Most organisations don’t have
With Data Stewards Day fast approaching I started to reminisce about the many data stewards I’ve had the pleasure of working with in the past. What struck me was just how many people take on the role of data steward - but under the guise of conventional roles. For example,
Dylan Jones (@dataqualitypro) shares an example of why data quality should be a business-as-usual practice.
Dylan Jones (@dataqualitypro) explains how data overloading occurs -- and how to find a better solution.
Blogger Dylan Jones offers 3 tips for more effective data profiling.
Dylan Jones (@dataqualitypro) explains why data migration should be a keystone of your data transformation.