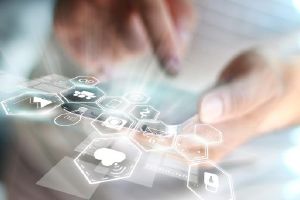
Omnichannel Analytics are helping companies uncover patterns in big data to improve the customer experience. Using those insights, companies can anticipate what consumers are planning to purchase and influence that purchase in real time. Companies are experiencing unprecedented complexity as they look for growth and market opportunities. Their product portfolios are