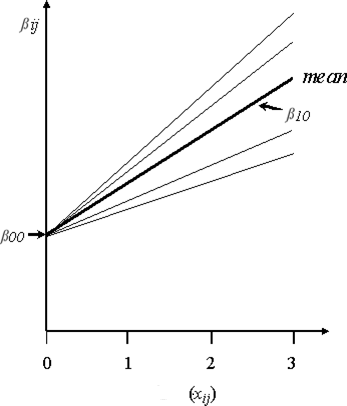
I recently received this interesting question regarding Multilevel Models after one of my last blog posts: Question: Can you tell me when a multilevel-model is not appropriate? I have data that by design is clustered but the random intercept in the null model is not significant. I have seen advice