SAS Learning Post
Technical tips and tricks from SAS instructors, authors and other SAS experts.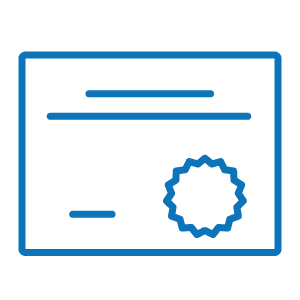
Updated Nov. 18, 2020 The demand for data skills has been growing at a rapid rate and will continue to progress for years to come. According to the World Economic Forum (WEF), Data and AI will experience the highest annual growth rate for job opportunities, at 41%. It’s no surprise
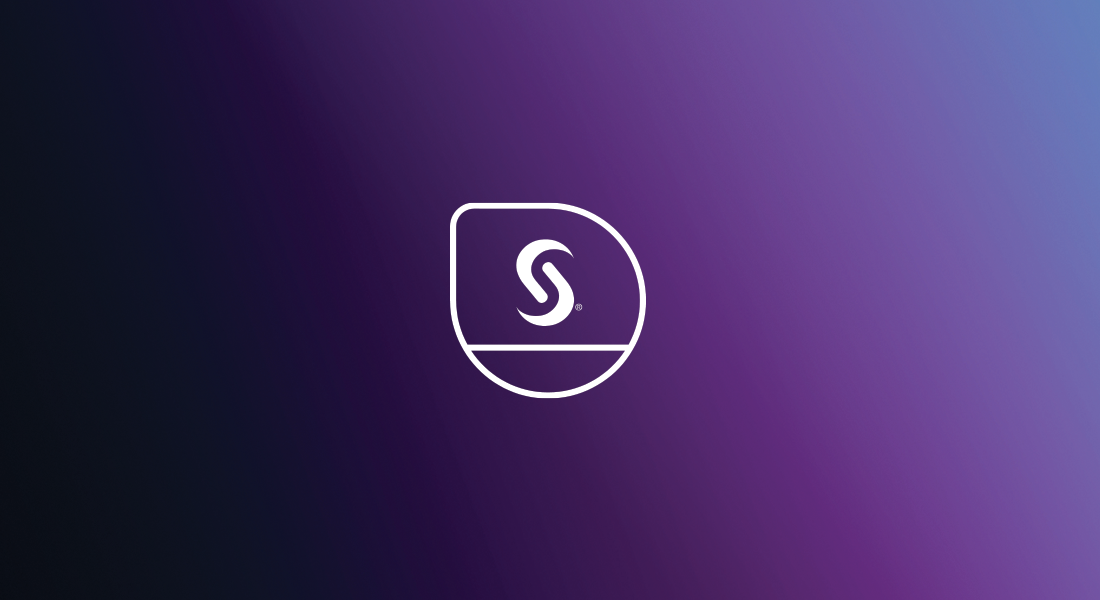
Preparing for the SAS Certified Base Programmer exam? SAS' Mark Stevens reveals the best ways to prepare.

SAS' Charu Shankar shares six tips for landing a job as a SAS user.
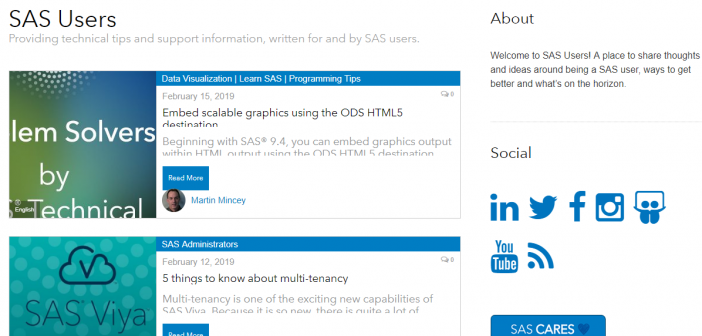
The SAS Learning post has merged with the SAS Users blog to provide you with all the training, certification, books, events, and programming tips you need.
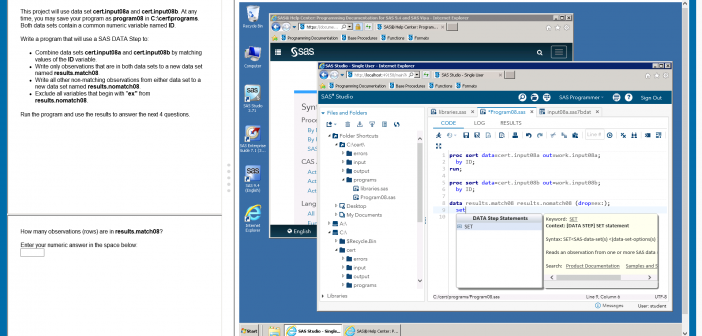
SAS has worked with our exam delivery partners to integrate a live lab into an exam, which can be delivered anywhere, anytime, on-demand.
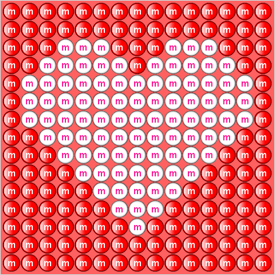