.@philsimon on the downside of the Band-Aid approach.
Tag: data management for analytics
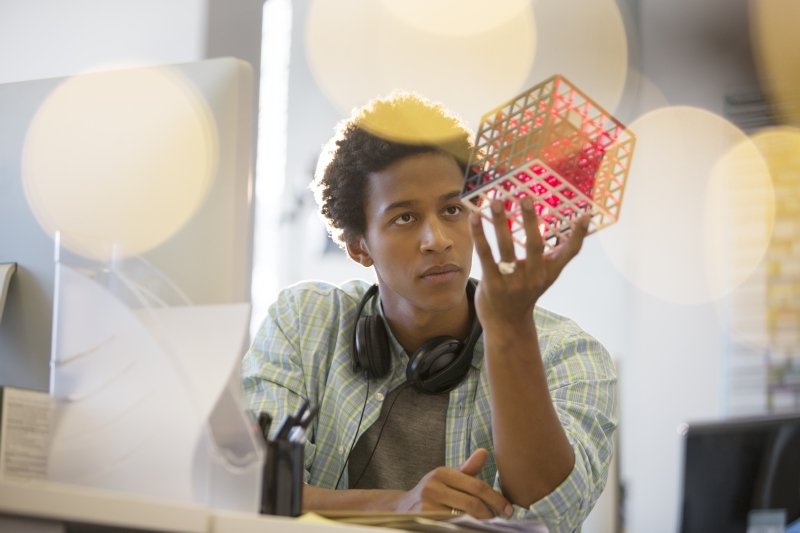
Critical business applications depend on the enterprise creating and maintaining high-quality data. So, whenever new data is received – especially from a new source – it’s great when that source can provide data without defects or other data quality issues. The recent rise in self-service data preparation options has definitely improved the quality of
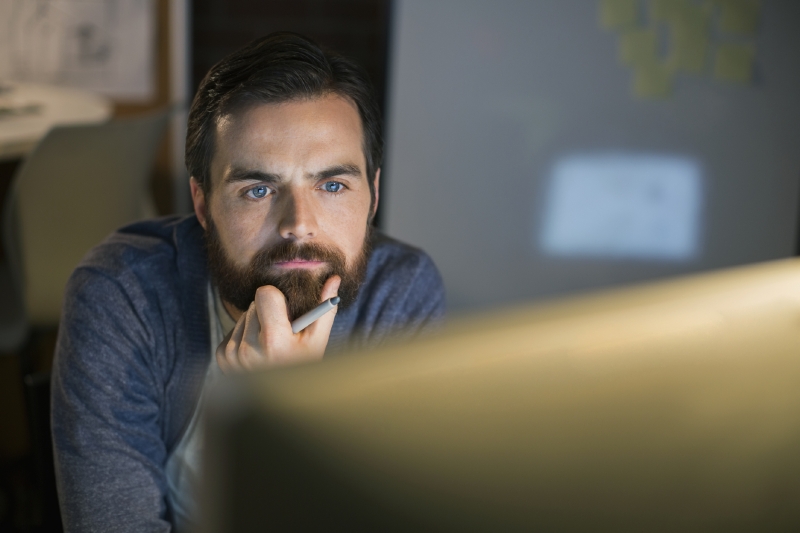
Hadoop has driven an enormous amount of data analytics activity lately. And this poses a problem for many practitioners coming from the traditional relational database management system (RDBMS) world. Hadoop is well known for having lots of variety in the structure of data it stores and processes. But it's fair to
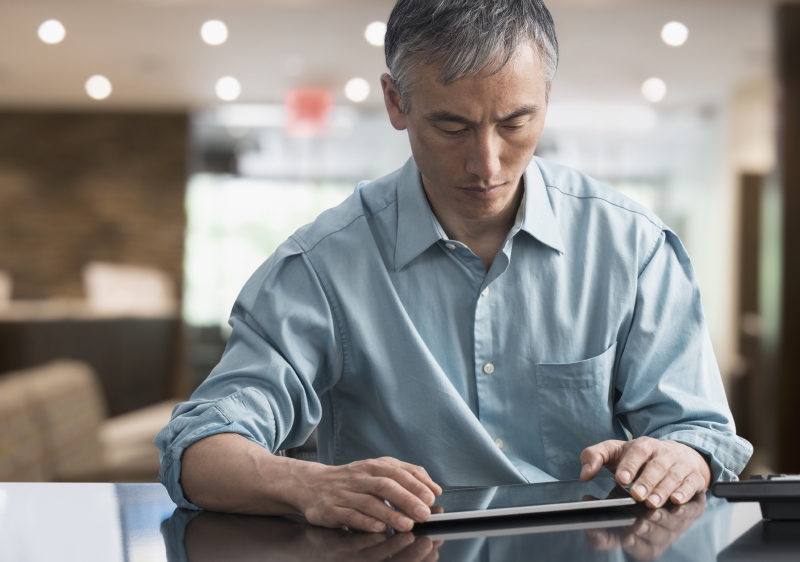
.@philsimon continues his series on data prep and anlytics.
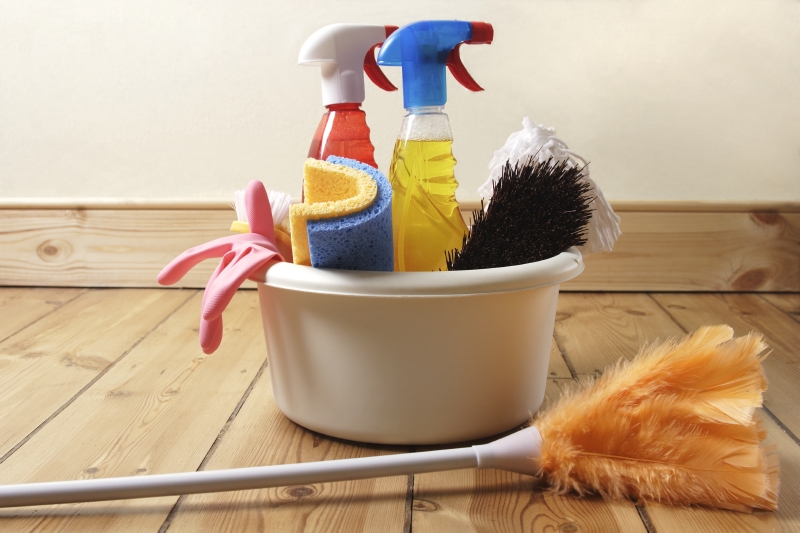
In my last post, I talked about how data still needs to be cleaned up – and data strategy still needs to be re-evaluated – as we start to work with nontraditional databases and other new technologies. There are lots of ways to use these new platforms (like Hadoop). For example, many
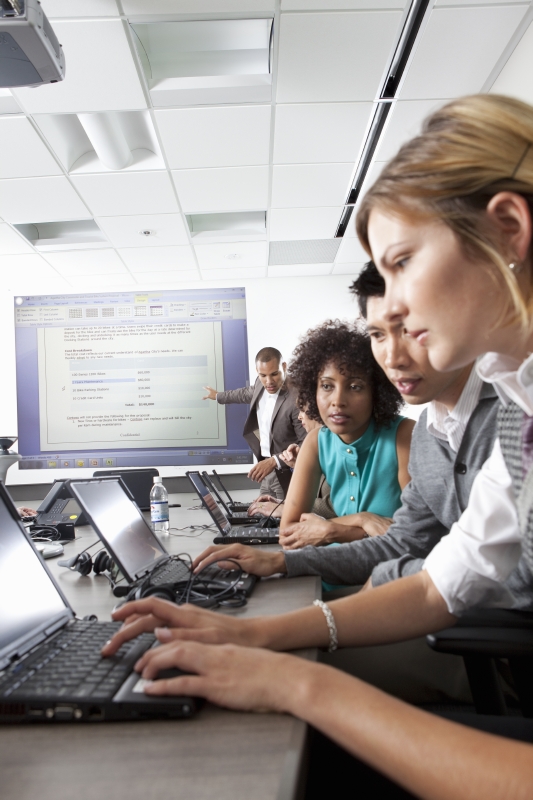
I'm hard-pressed to think of a trendier yet more amorphous term today than analytics. It seems that every organization wants to take advantage of analytics, but few really are doing that – at least to the extent possible. This topic interests me quite a bit, and I hope to explore
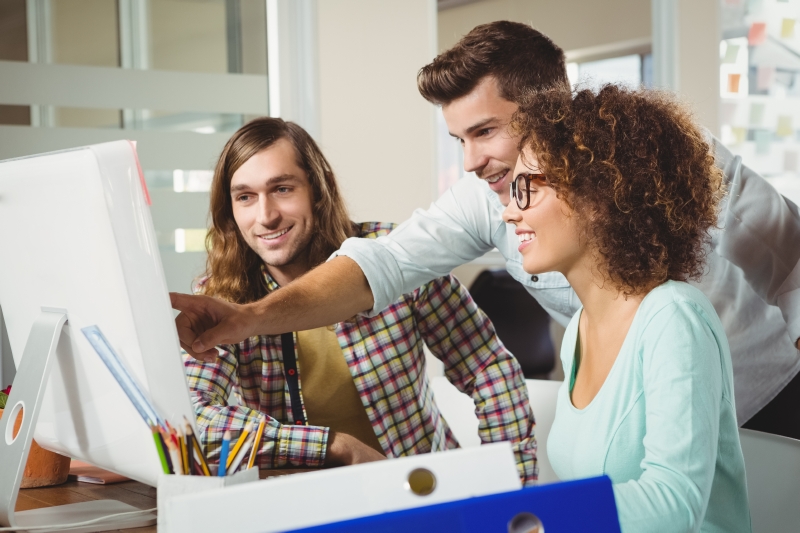
What does it really mean when we talk about the concept of a data asset? For the purposes of this discussion, let's say that a data asset is a manifestation of information that can be monetized. In my last post we explored how bringing many data artifacts together in a
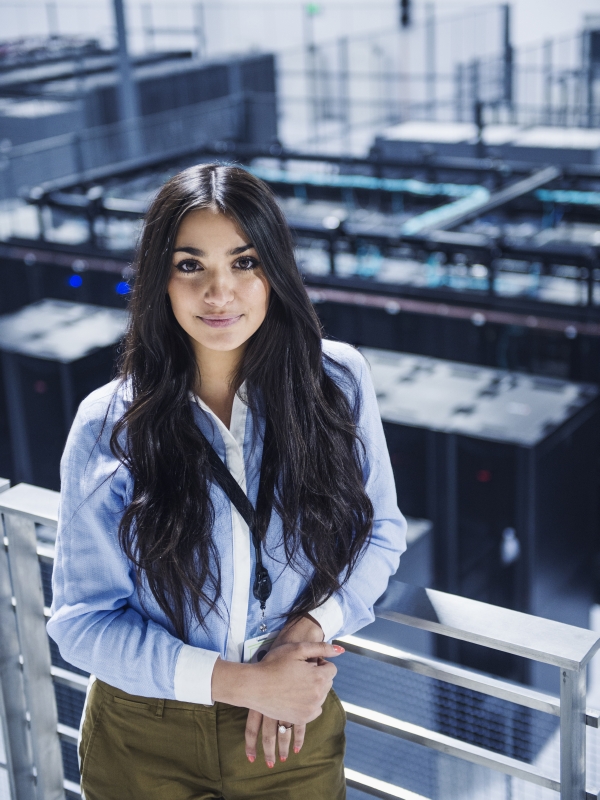
If your enterprise is working with Hadoop, MongoDB or other nontraditional databases, then you need to evaluate your data strategy. A data strategy must adapt to current data trends based on business requirements. So am I still the clean-up woman? The answer is YES! I still work on the quality of the data.
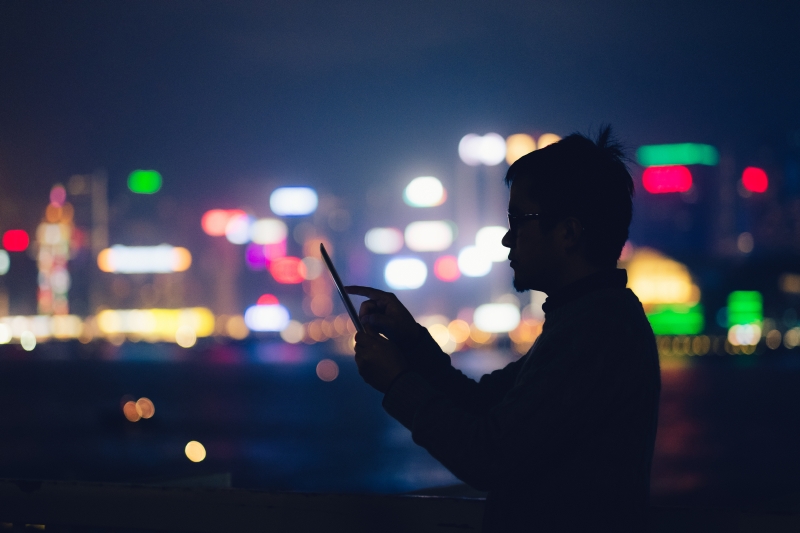
The demand for data preparation solutions is at an all-time high, and it's primarily driven by the demand for self-service analytics. Ten years ago, if you were a business leader that wanted to get more in-depth information on a particular KPI, you would typically issue a reporting request to IT
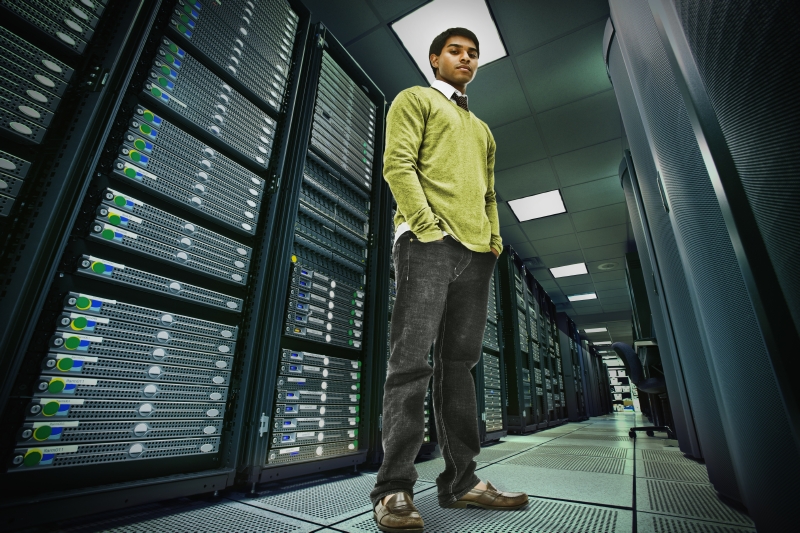
A long time ago, I worked for a company that had positioned itself as basically a third-party “data trust” to perform collaborative analytics. The business proposition was to engage different types of organizations whose customer bases overlapped, ingest their data sets, and perform a number of analyses using the accumulated
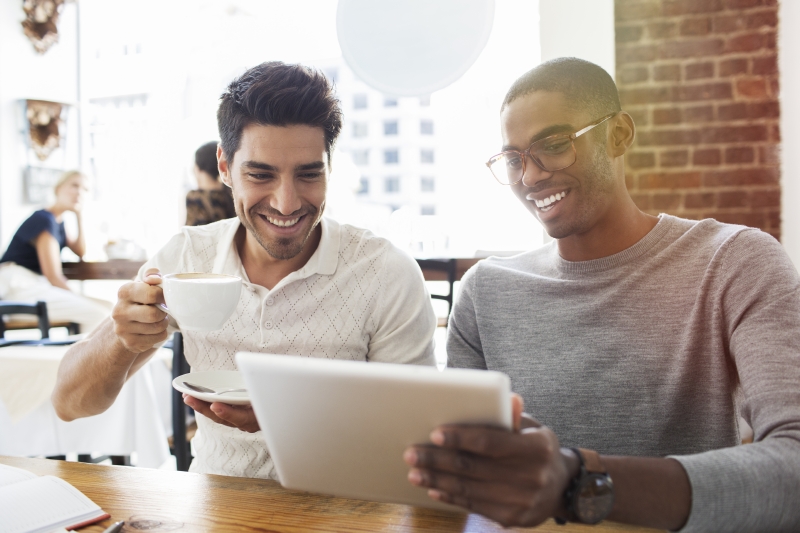
In my previous post I discussed the practice of putting data quality processes as close to data sources as possible. Historically this meant data quality happened during data integration in preparation for loading quality data into an enterprise data warehouse (EDW) or a master data management (MDM) hub. Nowadays, however, there’s a lot of
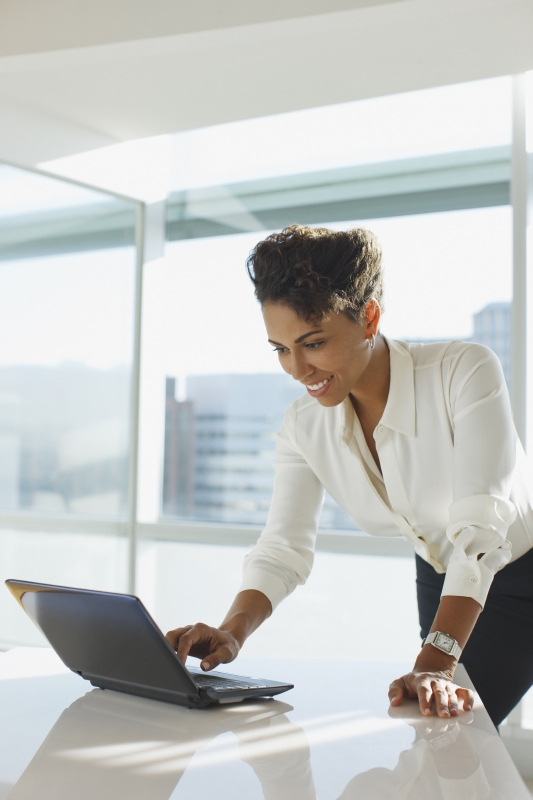
Throughout my long career of building and implementing data quality processes, I've consistently been told that data quality could not be implemented within data sources, because doing so would disrupt production systems. Therefore, source data was often copied to a central location – a staging area – where it was cleansed, transformed, unduplicated, restructured
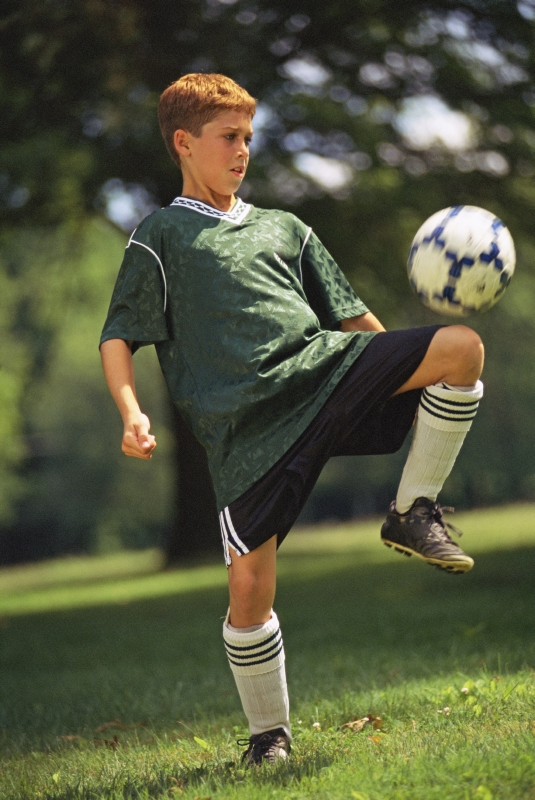
A soccer fairy tale Imagine it's Soccer Saturday. You've got 10 kids and 10 loads of laundry – along with buried soccer jerseys – that you need to clean before the games begin. Oh, and you have two hours to do this. Fear not! You are a member of an advanced HOA
(Otherwise known as Truncate – Load – Analyze – Repeat!) After you’ve prepared data for analysis and then analyzed it, how do you complete this process again? And again? And again? Most analytical applications are created to truncate the prior data, load new data for analysis, analyze it and repeat
Once you have assessed the types of reporting and analytics projects and activities are to be done by the community of data analysts and consumers and have assessed their business needs and requirements for performance, you can then evaluate – with confidence – how different platforms and tools can be combined to satisfy
In my previous post I used junk drawers as an example of the downside of including more data in our analytics just in case it helps us discover more insights only to end up with more flotsam than findings. In this post I want to float some thoughts about a two-word concept
@philsimon says that, yes, we can learn a great deal.
In April, the free trial of SAS Data Loader for Hadoop became available globally. Now, you can take a test drive of our new technology designed to increase the speed and ease of managing data within Hadoop. The downloads might take a while (after all, this is big data), but I think you’ll
In the last post, we talked about creating the requirements for the data analytics, and profiling the data prior to load. Now, let’s consider how to filter, format and deliver that data to the analytics application. Filter – the act of selecting the data of interest to be used in the
In the era of big data, we collect, prepare, manage, and analyze a lot of data that is supposed to provide us with a better picture of our customers, partners, products, and services. These vast data murals are impressive to behold, but in painting such a broad canvas, these pictures
One area that often gets overlooked when building out a new data analytics solution is the importance of ensuring accurate and robust data definitions. This is one of those issues that is difficult to detect because unlike a data quality defect, there are no alarms or reports to indicate a
What data do you prepare to analysis? Where does that data come from in the enterprise? Hopefully, by answering these questions, we can understand what is required to supply data for an analytics process. Data preparation is the act of cleansing (or not) the data required to meet the business
.@philsimon on what we can learn from Seattle's juggernaut.
In the last few days, I have heard the term “data lake” bandied about in various client conversations. As with all buzz-term simplifications, the concept of a “data lake” seems appealing, particularly when it is implied to mean “a framework enabling general data accessibility for enterprise information assets.” And of
As part of two of our client engagements, we have been tasked with providing guidance on an analytics environment platform strategy. More concretely, the goal is to assess the systems that currently compose the “data warehouse environment” and determine what the considerations are for determining the optimal platforms to support
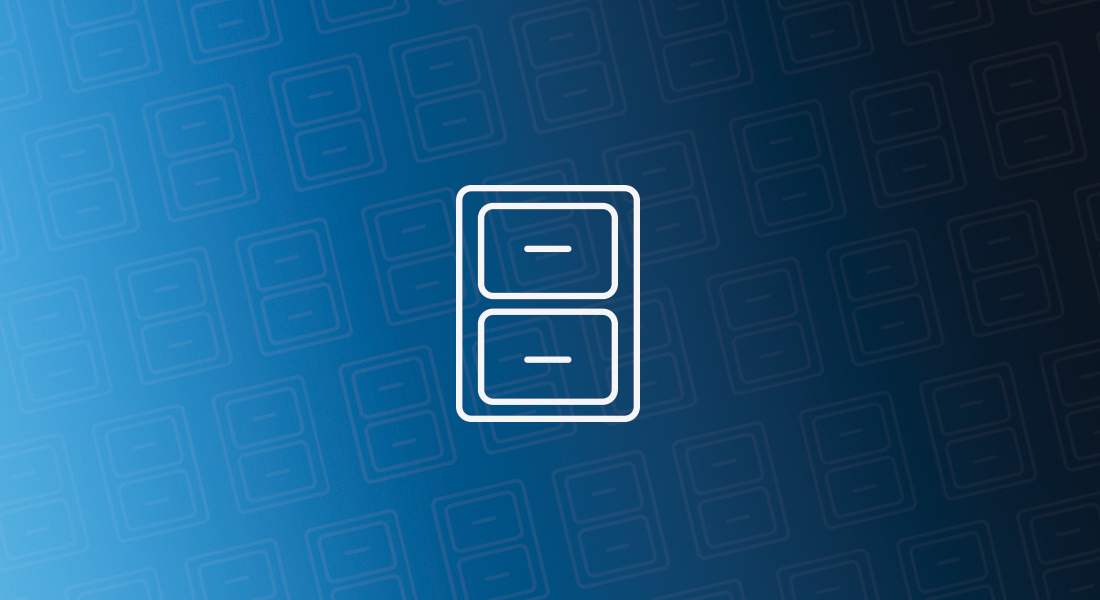
The adoption of data analytics in organisations is widespread these days. Due to the lower costs of ownership and increased ease of deployment, there are realistically no barriers for any organisation wishing to exploit more from their data. This of course presents a challenge because the rate of data analytics adoption
You may feel like the world is moving faster than ever. If so, then you can take solace in two facts: You're not alone in feeling this way. You're right. It is. Celebrating the 25-year anniversary of the Web, The Economist ran a piece examining the increasingly rapid adoption of new technologies.