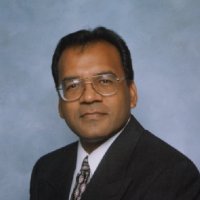
"Bad data does exist," says Sunil Gupta, SAS author and Global Corporate Trainer, Gupta Programming. Gupta is an expert in the pharmaceutical and medical device industry, but he volunteered this week to speak to an audience of SAS users in the insurance and financial services industries about minimizing the impact and risk of bad data. He believes that many of the processes, code and ideas used in the pharmaceutical industry can be applied across other industries with equal success.
Gupta began by showing examples of bad data encountered in the pharmaceutical industry (interestingly, these are also types encountered in other industries):
- duplicate records
- missing values
- start dates are after stop dates
- invalid values for variables
- poor quality data versus fraud data (trimming, cooking, altering or forgery)
"Hopefully these things don't exist in the financial world. It's always best to prepare for bad data and the reasons that cause the data to exist," Gupta said. He says there is a high price tag for bad data - poor decisions!
According to Gupta, there may be many causes for bad data including the data entry process, changes to the source systems (through acquisitions or mergers) or misunderstandings (perhaps with regulators or vendors). But there are great benefits to high-quality data - including elimination of 'interpretations' of the data across multiple departments. "If the data is organized - and not so much is missing - then you can come up with a single version of the truth," said Gupta. "That is very important."
Gupta said that limited resources prompted his organization rethink its approach data validation: Does all data need 100 percent validation? He called the concept "adaptive strategies." Of course, this strategy would have to be discussed with regulators. "This approach is totally approved by the FDA (regulators for the pharmaceutical industry)," he said.
Adaptive strategies:
- Start at 70 percent validation and increase or decrease percentage based on quality control issues found from the first clinical study
- Validate based on risk category - High (90 percent); Medium (80 percent) and Low (70 percent)
Another suggestion for all organizations is - take a proactive stance with your data. Gupta says the most important thing that you can do is to know your data. "You have to know what type of data to expect," he says. "Then you will know when your data is at risk."
Do you feel that these practices can be applied cross-industry? What other industries would they apply to? What other practices from the pharma world would work in yours?
2 Comments
Pingback: Bringing out your dead data: Data management challenges in higher education - SAS Voices
Pingback: Managing big data at the speed of risk - SAS Users Groups