The benefits of AI and machine learning do not present themselves without risks. From the trustworthy use of AI and machine learning to the ability to explain the workings of the algorithms to the amplified risk of propagating bias in decision making, internal and external stakeholders are rightly concerned. However, there are methods organisations can take to alleviate those concerns.
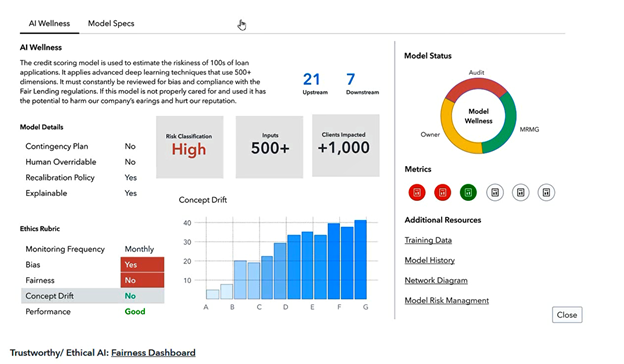
Defining bias and fairness in risk modeling
Bias in AI systems occurs when human prejudice becomes part of the way automated decisions are made. A well-intentioned algorithm trained on biased data may inadvertently make biased decisions that discriminate against protected consumer groups.
In risk models—including those leveraging AI and machine learning—bias may stem from the training data or assumptions during model development. Such data biases may arise from historical biases and how the data is sampled, collected, and processed. The training data does not represent the population to which the model will be applied, leading to unfair decisions. Bias can also be created during the model development phase.
Model assumptions can lead to measurable differences in how a model performs in definable subpopulations. For example, a machine learning-derived risk profile can be based on variables that approximate age, gender, race, ethnicity, and religion. It is illustrated by the historical racial bias captured by credit bureau scores. Although the scores do not directly consider race as a factor, they have been developed on historical data that includes elements like payment history, amounts owed, length of credit history and credit mix. The generational wealth influences these variables that African American and Hispanic borrowers did not have equal access to. Unless adjusted, the bias will continue to produce lower credit scores and lower ability to access credit for these groups.
Fairness, however, is considered a moral primitive and, by nature, judgmental. Given its qualitative character, it is more challenging to define fairness comprehensively and globally across applications. Distinct cultures will have different definitions of what constitutes a fair decision. When it comes to the technological approaches to incorporate bias and fairness checks in AI systems, in recent years, AI and machine learning software providers have started to package detection and remediation techniques.
In the USA, fair lending laws, such as Regulation B and the Equal Credit Opportunity Act (ECOA), protect consumers from discrimination in lending decisions. The statute makes it unlawful for any creditor to discriminate against any applicant concerning any aspect of a credit transaction based on race, skin color, religion, national origin, gender, marital status, or age. A good starting point to assess fairness is by comparing the predictions and the performance of a model or decisions across different values of protected variables.
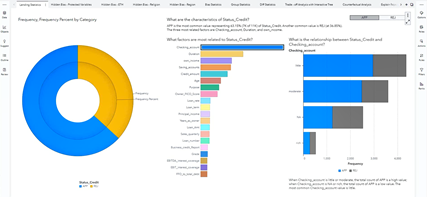
Methods and measures to address fairness
Today, a range of metrics and methods exist to assess the fairness of model outcomes. For risk management, it is recommended that bias and fairness checks are embedded as controls throughout the model lifecycle at the data, model, and decision layer. It is also important to understand the limitations of fairness metrics: the measures to detect fairness risk cannot guarantee the presence or absence of fairness or prevent it from appearing later due to exogenous factors, such as changes to data or policy changes. Some popular metrics to detect fairness risk that can help signal for human intervention to correct it are explained below:
- Demographic parity index: This is where each group of a demographic variable, also referred to as a “protected class,” should receive the same positive outcome at an equal rate.
- Equal Opportunity: The metric confirms that the actual favorable rates between groups are the same. By extension, comparable, accurate negative rates are seen across groups.
- Feature attribution analysis: Feature attribution analysis finds the key drivers that affect model or decision outcomes.
- Correlation analysis: Correlation analysis assesses the correlation between critical drivers and protected variables.
- Positive predictive parity: Here, the favorable predictive rates across groups of protected variables are equal. This is achieved by comparing the fraction of true positives to the fraction of predicted positives in each group. The parity allows for the measurement of the distribution of benefits across groups.
- Counterfactual analysis: To assess fairness at the individual level, the counterfactual analysis compares the causal attributes of the same record with an adjusted version of the record to evaluate the change in outcome. Considering all else equal, with a change in the value of a protected variable, such as race or gender, do we see a difference in the model or decision outcome?
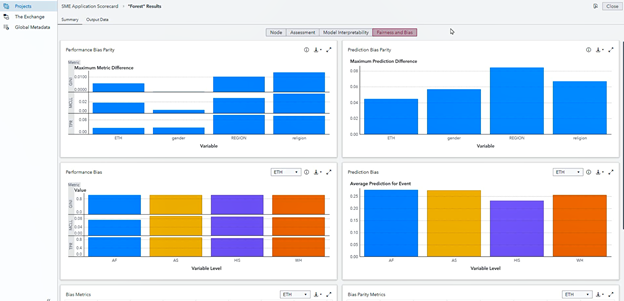
Measuring bias and fairness can create more effective risk management
Effective risk management is increasingly being brought to the frontline rather than functioning in the back office. When using advanced analytics, it’s becoming increasingly important to understand and measure fairness risk to avoid exploiting vulnerable consumers. Creating frameworks and processes to mitigate bias and address fairness risk will mean that it can be expanded to other risk models with rigor in the future.
1 Comment
Great article, Stephen! Happy to see your contribution to SAS and customers in this space 🙂