I have just completed an MSc in artificial intelligence and have been reflecting on my key takeaways from this experience. Study and learning like that is intrinsically interesting. And all the more so when everyone around you talks about analytics and artificial intelligence. Many would argue that it is worth doing in its own right. However, I think it is also important to consider how we integrate our new skills and knowledge into our "day jobs" and how to correlate everything in an architectural aproach.
My day job as a manager and architect is to guide customers on their architecture. Like many others in the same role, I often find myself mediating between business units and IT. The pressures and requirements on the two sides are very different. Business units want everything rapidly – especially with the speed of change that has been driven by the pandemic. They also want built-in flexibility to scale up or down to meet demand.
IT units, however, need to know that everything has been done properly and without compromising security and governance. Speed and flexibility are inherently dangerous because they encourage people to cut corners. But one of the most important aspects is seeing the big picture of the ecosystem and what difficulties may appear in advance, technical or not. When this includes an exploration of how analytics and artificial intelligence participate in enterprise architecture, it is a very interesting exercise.
When I combined my studies with my day job and passion for architecture, I saw four important crossovers.
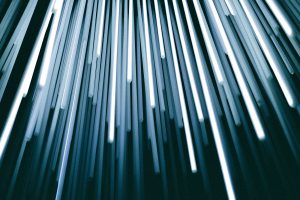
1. AI is not magic
There is a tendency for people to believe that AI is some kind of magical solution to any problem. The huge amount of hype about AI has convinced people to think that it is the answer to everything. This means that if what you are suggesting does not involve AI, then it can’t be the right answer. However, AI is not magic. It is all about data, maths and statistics. And as with any other maths and statistics, it is good practice to use the simplest possible method to deliver the required results.
Both IT teams and business units may find this hard, especially if they are not analysts. Architects have the professional authority to resolve this difficulty and the responsibility to make everyone understand that maybe it is worthless to change an architectural approach just for one case that is not even clear in terms of business outcomes. As an enterprise architect in an analytics company, we should be able to explain the basics – such as the SGD approach, for example – to a business stakeholder and explain the consequences in terms of technical and business costs. The best solution does not have to be the optimal one.
2. There are many fields where AI is not proven
Related to the idea that AI is not a magic bullet, it is also unproven technology in many fields, so it is always good to have a critical point of view with it. Even in sectors and industries that already widely use AI, there may be areas that have not tested it. That does not mean that AI is not applicable, of course. New use cases are being developed all the time. However, my role as an architect is often to help business units to understand that there is a certain amount of risk in adopting unproven solutions.
It may be better to use a proven technology before looking to the cliff. This is especially true if the results of the analysis are business critical. There are many ways in which AI can go wrong, not least in what it learns inadvertently from the data. It’s funny to see how everybody cares about the algorithm and the AI technique to be used, instead of wondering if they have the correct data to extract the information that AI needs to work.
3. Where is the crossover between AI and enterprise architecture?
As advanced analytics, including AI, has become more essential to businesses, it has also become an increasingly important part of enterprise architecture. Enterprise architects are responsible for planning IT capabilities across the organization. Increasingly, that needs to include analytics systems and platforms. In other words, AI, or the potential to use AI, needs to be part of the architectural vision for the enterprise. The use of a good analytics platform can create that flexibility by enabling additions of new technology and scaling capacity. The analytics architect’s role is to work with enterprise architects to ensure that the overall IT vision has space for analytics and AI.
Advanced analytics and AI cut across the four essential horizontal layers in EA. Of course, the business with the process to get value, application and data inherently in software and data architecture. And, of course, in a technical infrastructure plane in the way to deliver the solutions. But not only in horizontal layers but also vertically across planes such as security, governance and motivations.
Could we think in a new dimension in an enterprise architecture landscape for AI? This could be a good point to explore in another article.
4. Remember ethics in AI
AI comes with huge ethical issues and responsibilities. Despite the theory, AI-based algorithms are not neutral. They learn what we teach them, which means that our unconscious biases tend to be transferred to the algorithms we train. During the early development of facial recognition software, for example, the algorithms struggled to distinguish black and minority ethnic faces. The developers – overwhelmingly white men – had used existing picture libraries and inadvertently trained the algorithms to recognize white people.
AI-based models can also become black boxes. This is a problem because we need to understand the basis of the decisions made by these models. There is a balance to be struck between explainability and accuracy. As algorithms become more complex and autonomous, we need to be sure that we are not creating monsters. And, above all, that we understand the overall context and not only the lab world.
Architects are among those charged with helping to make these tradeoffs and decisions and also to ensure a common and understandable language, tailored if needed to the stakeholder we have in front and to communicate from business through infrastructure without creating our own speech with the fancy words everybody is expecting to hear.