Over the past few years, digital transformation has finally started delivering on its promises. Many financial and commercial institutions have made significant strides in supplementing their traditional customer service channels with user-friendly digital equivalents. Meanwhile, a plethora of innovative startups has shaken up the banking, insurance and retail markets by offering digital-only services, typically centred around powerful mobile apps.
As a result, among younger demographics in particular, there’s now an expectation that digital is the default – and anything that can’t be done online is seen as a major inconvenience. But while digital services make it easier for customers to interact with your business, they also make it easier for fraudsters to rip you off. Online channels are an open invitation for cybercriminals to attack your systems, abuse your processes and undermine your security.
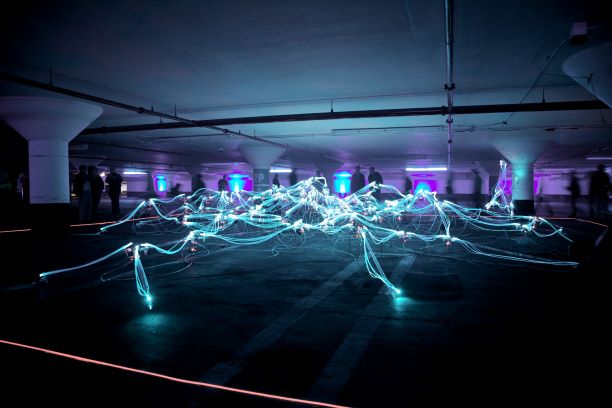
Accelerating the shift to digital
In the early months of 2020, we have seen a boom in digital services, while the traditional physical economy has slowed to a crawl. To stay in business, many companies are being forced to move services online faster than they had planned. In the rush to get these new digital services to market, there’s a significant risk that development teams will make mistakes and overlook the usual security checks. Unfortunately, the likely result is that fraudsters will have a field day as they find and exploit these new gaps in their victims’ armour.
Need for speed in fraud solutions
In a highly dynamic environment where fraudsters are discovering new attack vectors every day, it’s critical for fraud prevention teams to be able to detect threats and respond quickly. Artificial intelligence and machine learning (AI/ML) approaches can help by spotting patterns in previous fraud cases and using them to detect suspicious behaviour by customers, employees or systems.
AI/ML is a vast and highly technical field, and it can be difficult for fraud teams to choose the best way to start their adoption journey. Nevertheless, at SAS we’re already seeing banks and other organisations put a variety of interesting AI/ML-powered anti-fraud solutions into production. For example:
1. Computer vision
Digital banks such as Monzo are using smartphone cameras with facial recognition technology to prevent unauthorised users from gaining access to customers’ accounts via their mobile apps. Today’s powerful facial recognition solutions are built using machine learning models that can tell the difference between a customer’s face and a photo or mask. They can even detect when a person is sleeping or unaware that the camera is being used, potentially making them a much more powerful access control measure than traditional password-based login methods.
Banks are also using image recognition to streamline processes such as paying in cheques, where customers simply take a photo of the cheque and upload it via their banking app. Banks already use machine learning models to identify whether the image is a genuine cheque and extract the key information from it. It will be a natural progression to analyse signatures and detect more types of potential cheque fraud.
2. Natural language processing
Natural language processing and text analytics can help companies handle larger volumes of internal and external communications – such as phone calls, emails, SMS and instant messenger/chatbot interactions – while still maintaining robust anti-fraud measures. For example, in a banking context, many institutions already record the phone calls of their traders and other employees to provide evidence in cases of insider trading and other financial crimes. By using natural language processing techniques, organizations can automatically transcribe these audio files into text. Then AI/ML models can recognise relevant keywords and topics, analyse tone and sentiment, and raise alerts to the fraud team when suspicious behaviour rises above a given threshold.
3. Reducing false positives
False positives are the bane of fraud investigators’ existence, diverting expert resources away from the true criminals and alienating innocent customers and employees. You can use AI/ML techniques to build models that can analyse previous cases and separate out the behaviour patterns that are truly suspicious from the purely superficial anomalies.
4. Optimising rule-based fraud detection
Many current fraud detection systems use a defined set of business rules to assess the likelihood that a given case requires investigation. You can use AI/ML models to supplement and test these rule sets. This provides insight into the relationship and relative predictive power of each rule and even suggests new rules that can be added to increase the accuracy of the results.
5. Identifying collusion
One of the most powerful tools in an investigator’s toolkit is network analysis, which provides tools to visualise and understand the relationships between the people, places and events surrounding a case under investigation. Just like human investigators, AI/ML models can be trained to interpret these complex networks, and can often identify patterns and relationships that traditional approaches might miss.
6. Network monitoring
The move towards providing digital services for customers and remote working capabilities for employees poses new problems for network security teams, who can no longer count on all sensitive activity taking place behind the corporate firewall. However, you can also use AI/ML solutions to process vast quantities of network logs and identify suspicious events at a speed and scale far beyond the capabilities of human network administrators.
Putting a platform in place
Many companies begin their AI/ML journey with open source tools, and that’s a valid approach for prototyping and small-scale deployments. However, scaling up with open source technologies is hard – at an enterprise level, you really need a more robust approach. At SAS, we’ve seen clients succeed when they take a centralised approach. This means adopting an analytics platform that can support both traditional statistical methods and AI/ML techniques. You also need governance to ensure the appropriate use of information, proper model testing and monitoring to avoid inaccuracies, and bias mitigation.
If you’d like to learn more about current trends in risk and fraud and plan your journey with AI/ML, listen in to our free webinar, Managing Risk in the Age of Fraud. And if you’d like to discuss any of these topics further, feel free to reach out to me on Twitter or LinkedIn, or email me at caroline.hermon@sas.com.