I recently chaired a roundtable discussion focused on fraud and security intelligence at the 2019 SAS UK Forum. The session involved over 20 people, all with differing perspectives, views and experience. It was a great way to explore the topic. Obviously, there were a number of differences between individuals and organisations, not least in approach. However, there were also a surprising number of similarities between the participants, and I think these may show some ideas worth developing in future.
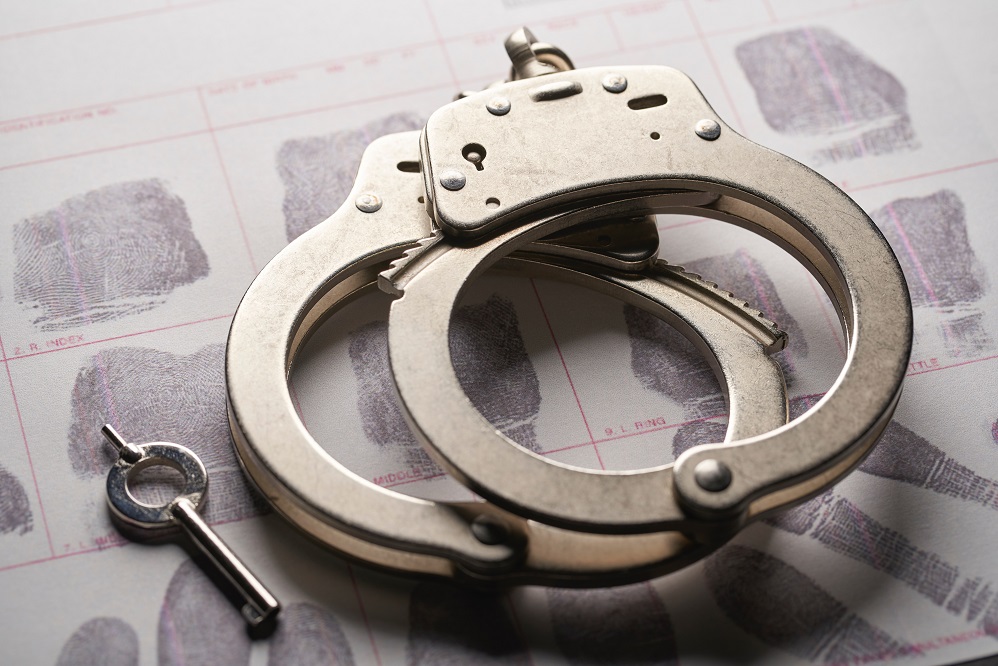
Fraud detection and credit decisions
Analytical techniques can describe groups and individuals at various levels of granularity to support an automated and informed decision. Analytics supports the process of asking questions such as "Do we believe this transaction is an account holder authorised activity?" or "Do we believe, given the history of the individual, we should extend credit lines and repayment be made if we do?" Using artificial intelligence means that you can also answer the "unknown" questions – the ones you didn’t know you should ask. The answers can be used to suggest what action should be taken next.
Correct identification is a key part of the business process
The core process relates to risk identification. Authorisation of transactions is still key, whether this relates to an application for extended credit facilities or highlighting a potentially fraudulent transaction. The background activity is based on core advanced analytical techniques.
Identification comes with some very specific challenges – mainly centred around volumes and quality. Too many cases highlighted have operational impacts. A high volume of potential fraud cases requires a larger investigations team and supporting process. Too many credit applications declined can affect revenues. Both similar sides of the same coin.
Identified fraud cases must be of the correct quality, and credit lines refused for the appropriate risk reason. These are both dynamic situations changing throughout the business day, so also having the ability to alter and adjust quickly is a significant business advantage. This is a core application for advanced analytics.
Ongoing and continuous monitoring, coupled with better use of advanced analytics, is a good approach for spotting suspicious patterns at very early stages.
Getting it right – on time
Timeliness of action was a further area for discussion. This had two angles to consider. Deciding where to start, if the work queue was significant – top, middle or bottom? And how then to identify if things were going awry in the process – so perhaps changes in the market or systems were causing issues.
Starting in the work queue in the wrong place could mean missing the opportunity to intervene before there is a problem. There may be cases with time constraints. Or delay could allow problems to affect critical business processes. However, failing to take enough time could lead to too many false positives.
Drilling into the investigation process identified additional differences relating to data provenance and ownership. In particular, different organisations had differing requirements relating to source and management of data as part of the process, or how to produce evidence for judicial proceedings.
However, there were also significant similarities – and they suggest changes that might be important in future. For example, most organisations report a significant reliance on the knowledge of members of the investigations team. They needed to know about data systems and workarounds and also have a very good network of personal contacts to gain access to data. The discussion explored how this approach is dangerous, perpetuating poor data collation processes and exposing frailties in data provenance. The potential impact would be a reduction in the efficiency and effectiveness of the investigations team.
A focus on business, not technology
There were a number of lessons from the roundtable. First, the problems were similar across sectors and networks. We can learn from each other, and more sharing of cross-industry examples would be useful because we are all targeting similar behaviour.
Fraud detection is a wide field. Every organisation experiences the implementation differently. Collin Bristow shares his experiences with us. Click To TweetThe second lesson is that everyone wants more good quality data. This can be used to speed investigation and create insights, such as automated network generation.
A third lesson is to consider business entities – so a phone number, for example. This is different from a customer, but can, when linked with automated networks, identify insights that had not previously been considered or seen.
The fourth lesson relates to the end game – enterprise-level investigation functions (crossing lines of business or department). Politically it still seems these are still a long way off.
Finally, there was one big question: How do we maximise defences and efficiencies against fraud, but minimise customer friction? There are no easy answers; it is very much a balancing act.
SAS plans to continue roundtable sessions because similarities across industries and shared knowledge both help to propagate innovation. Let me know if you would be interested in taking part in future events. Just make sure you come ready to explore, expand and share.