Anyone who might have stumbled across my previous musings will already know that I am a great fan of studying the history of technology and its evolution. And where we might indeed be in the not too distant future.
I’m of course a proper sci-fi nut, avid (and my wife would say, fairly addicted) video gamer and an AI optimist. I am also someone who is lucky enough to work in computing and analytics, something that if I did not do for a living, I would be doing anyway as it has been my main hobby since the age of 11.
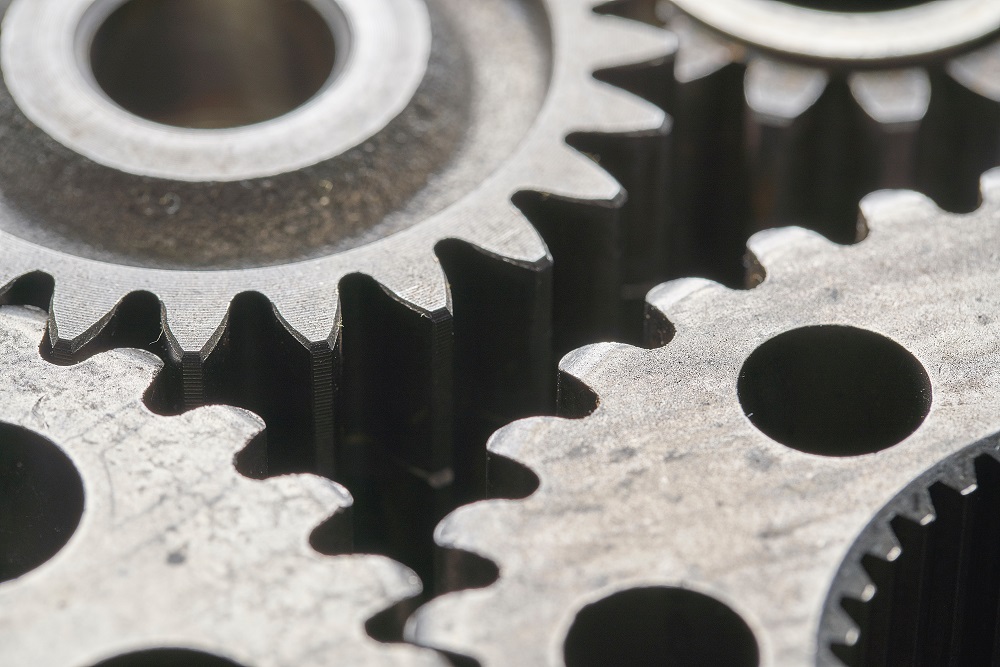
Motivation as a key factor?
While evaluating innovative projects that SAS gets involved in, I often think about motivation, the key factor that drives each and every one of us in everything we do.
To me, this is an evolved trait. Over centuries of development and learning, we have evolved the built-in capability to seek out new ways of achieving and refining processes and techniques. We discard those that were once the cutting edge but now prove outdated or inefficient. For example, we might replace a map in the car with a GPS receiver. It’s no surprise that in nearly every new major step forward that we take, we are inspired by what we observe around us.
Recently I was reading up about the dawn of human flight, partly because of the recent advancements in drones, such as taxis (more info here), but also because of the 50-year celebration of the first manned lunar landings. And I stumbled across a letter by Orville Wright which I partly quote for you here.
Now birds come in
What I find so appealing about this particular extract is not only does it make obvious to any reader that the origins of successful human flight come in part from the observation and study of naturally evolved bird flight, but also that the activity Orville observes demonstrates that the birds themselves have enhanced their evolved skills of flight by working together to effect change in the environment, allowing them to gain height and soar. Essentially, the birds were motivated to work together to gain collective advantage from their flying skills.
This leads me once again back to the essence of motivation and how it, in turn, drives advances in evolution. Let’s first examine AI. To train any type of AI, narrow and specialised or wide and general, you need data. In fact, you need a lot of it. The wider and more general the AI you are trying to create, the wider and bigger the data source you will need to feed it with.
Harnessing the IoT
If we look at the most abundant sources of data that are available to us today, we can really only get that data from one place, the IoT. This is where, according to our partners at Cisco and many other reports, the number of connected devices generating data all the time will exceed 50 billion in 2020. If we multiply that by the average number of messages per second, they can all generate which is thought to be around 800 terabytes per second at the time of writing this (see here).
We come up with a number so large it hurts my brain to try to think about it: 1,000,000,000,000,000,000 bytes per day, or simplified, about 1 exebyte (see here). So the data we need to build AI is there – we just need to start assessing it. The challenge is in solving the age-old problem of where to put it all so we can start to make sense of it. Or, rather, gain intelligence from it.
Luckily SAS and our many partners around the world have been working towards catering to this for some time. In fact, IoT Evolution World recently named our SAS Viya based analytics for IoT a 2019 Product of the Year. We have also been very busy working with our partners large and small to expand the footprint of IoT and cloud platforms we are integrated with (we are even white-label embedded inside some of them 😊).
Skills & knowledge are acquired
We are motivated to do this because of the skills and knowledge we have acquired over the last 40-plus years as a market-leading analytics company. And we know that the best way to evolve AI and IoT is to work in collaboration with other best-in-class partners and platforms. But no matter how much space or compute power we allocate to processing the data for AI in the cloud, there will always be too much to centralise it all. This is the reason for the emergence of edge analytics.
Edge analytics
Edge analytics (or more widely, edge computing) is basically a way to collect, assess and analyse data right out at the edge, where source devices generate the data, maybe even on the edge device (such as a camera) itself. This can, in theory, allow us to train and then put AI models directly on small, operations-critical equipment. This means that we only have to stream the intelligence derived from that data to downstream or central systems for action to be taken instead of all the raw data generated (conveniently that also describes the core principle behind streaming analytics). By doing so, we are enabling the right analysis in the right place to derive the best value for the need at hand. And SAS can then manage, deploy and monitor the analytics models (even if they're not built in SAS).
It’s a really exciting time in the evolution of #AI and #IoT (or #AIoT, #artificialintelligence of Things) since it’s the partnership of the two that is already beginning to revolutionise the way we work. Click To TweetIt’s a really exciting time in the evolution of AI and IoT (or AIoT, Artificial Intelligence of Things), since it’s the partnership of the two that is already beginning to revolutionise the way we work, live and play. And in time, perhaps, our descendants will look back on this stage in the evolution of technology as a key moment in our own evolution, just as we now look back on the pioneers of early flight, like the Wright brothers.
Note: REF Orville Wright letter quoted from here.