Beyond the wow around artificial intelligence is the reality. If you don’t use AI to make actual business decisions, it’s probably a waste of time, effort and money! From recommendations for your playlist to the next-best offer to decisions about customer targeting and company strategy, we are all looking for better decisions. But is it a straight swap with existing mechanisms, or will the scope of decisions also change?
Thinking about change
In a recent report published by UNESCO, Pierre Balloffet of HEC Montreal suggested that there could be three ways in which AI could affect us. As a mathematician myself, I could appreciate the way he described them: addition, subtraction and multiplication.
Under the subtraction scenario, AI would either take over or reduce the value of human input into a wide range of jobs, including professional roles. The result would be far-reaching job losses and consequent social change.
The addition scenario is more optimistic, suggesting that AI will never be more than a decision support tool, however good it gets. It could, however, help us to make much better decisions across a wide range of areas. This is, more or less, the current state of play; AI is helping to improve decisions in a variety of fields, including health care, client relationships, and choosing books or music. But it is only a support for what is, ultimately, a human decision.
The third scenario, multiplication, is quite different. It suggests that bringing together people and machines could result in a massive increase in the abilities of both. Working together will augment, or multiply, ability. However, Balloffet notes that in this scenario, it is important to remember that we might need more than AI. He suggests that connections will be the crucial element, rather than the precise nature of the technology.
Under these scenarios, does the scope of decision making change? Certainly, under the addition scenario, the implication of making better decisions is that a decision has to be made. When we have more information, however, the potential options may also expand.
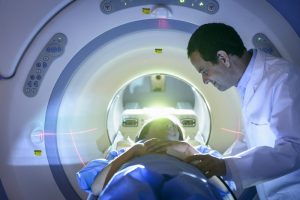
Consider health care, for example. Once a diagnosis is made, there are options for treatment, and doctors must decide between them. With more information, doctors will know more about which treatments may work better for which individuals, and the likely effects of each option. In some ways, this shuts the decision down, because it makes the choice obvious. It may, however, also open up more personalised treatment options, or even show that no treatment is going to work well. Either way, the scope does change.
Quality of training makes a difference
How will AI work to help make better decisions? I’m often presented with the argument that all AI could do is to lower the cost of prediction. This, however, has significant implications. If the cost of relatively accurate prediction is lower, it can be used more often, and to help with more decisions, and particularly more complex decisions.
Quality of training makes a difference in making better decisions with the help of #AI Click To TweetArguably, this is where good model design discipline can come into play. First, we need a way of deciding whether AI could help with a particular problem. It is a good starting point as a way of working whether we have the necessary information. The framework starts with the target hypothesis; what do we need to predict to answer your question? Next, we need to apply judgment to place a value on different outcomes. The third step is to specify what we are trying to achieve, then find metrics that will tell us whether you have been successful. These elements describe the aspects of the decision that we need to make. Thus far, no different from what we do today.
The next elements are about the data that we need. First, what input data is necessary, and is it (or could it be) available? How do different data points interact with each other to potentially give more insight, or conflicting insight? How will we train the algorithm? This, too, will require data, but may be cruder than the planned input data because historical data may not be as complete or as accurate. We then also need to determine how the outcomes will be deployed and monitored into a decisioning framework – utilising the plethora of channels, technology and methods available today and in the future. Finally, we must consider how we can use feedback to improve the algorithm’s decisions over time. This is particularly important if our training data is crude.
The opportunity becomes more nuanced when real-time data is available. While real time may not always be right time, it does present us with compelling opportunities for better decisions if the context is right. Algorithms that can be trusted to learn can make a huge difference.
Redefining decisions
This framework can help to identify problems where AI might help to improve decision making. In a neatly circular way, it is a way to make better decisions. Decision-making tools come in multiple shapes and sizes; it is only the technology that varies.
The bigger challenge will be in helping humans reimagine the types of decisions that AI could eventually support. Analytics is already transforming the way decisions are made in government, for example. While the capability of analytics has made it possible, the catalyst was imagination to “dream up” use cases to solve pressing issues. With AI, imagination will be even more critical. Machines and humans will need more training. Machine learning is one thing – human learning is, in my experience, a bigger challenge!
Laurie Miles’ blogpost was inspired by “How AI Will Change the Way We Make Decisions” by Ajay Agrawal, Joshua Gans and Avi Goldfarb. Find the original article, as well as others about important aspects of AI, in the Harvard Business Review report “Risks and Rewards of Artificial Intelligence.” Download your copy here.