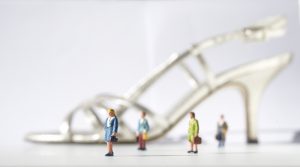
What size does an organisation such as a bank need to be before it can deploy analytics in earnest? The answer now is, surprisingly small. Analytics is no longer the preserve of big organisations. More and more small and medium-size businesses, including banks, are starting to adopt advanced analytics and machine learning, and seeing the benefits of decisions driven by data rather than instinct alone.
Using analytics in banking
In banking, the size of operations is far less important for analytics and machine learning adoption than the fact that many business processes now need advanced analytics to enable better decisions. Analytics is being used successfully to improve processes such as assessing loans and deciding which customers are the most likely prospects, as well as the conditions to offer for loan repayments. Banks are also using analytics to define new customer segments and develop possible products for them, with better certainty about the likely customer reaction.
In banking, the size of operations is far less important for analytics and machine learning adoption than the fact that many business processes now need advanced analytics to enable better decisions.
Analytics is also useful in detecting fraud, including from customers, employees, suppliers and other stakeholders, and rapidly counteracting it. It has even made proactive fraud prevention possible by identifying those most likely to be at risk of committing fraud, and taking steps to stop them. These issues are important for any bank, and large numbers are now finding that they cannot easily continue to do them effectively without analytical support.
There is, however, an issue with this – and that is cost or, rather, affordability. Organisations with small to medium-size technology budgets will not be able to afford or manage the computing power required for large-scale analytics in-house. What’s more, this size of organisation often struggles with the idea of making a large initial investment in technology that has not yet been tried and tested within the company itself. They frequently prefer to be able to start small, and scale up as demand and capability increase.
Finding solutions
Fortunately, there are increasing numbers of possible solutions to this dilemma. Cloud analytics is one option. Gartner describes cloud analytics as any analytics initiative in which one or more of the six elements – data sources, data models, processing apps, computing power, analytical models, and sharing or storage of results – is carried out in the cloud. Examples of cloud analytics include, therefore, software-as-a service analytics applications and business intelligence, and hosted data warehouses. Software-as-a-service is particularly useful for those organisations that need to scale up over time because additional capacity can simply be accessed when needed.
The great benefit of software-as-a-service for business intelligence and analytics is its flexibility. Not only can it be scaled up or down to match demand – a huge benefit to organisations on a tight budget – but the actual software available to users can also be changed to match demand. You can choose packages that are all-singing and all-dancing in analytics terms, or simply opt for something that allows users to do some visual analytics and a bit of data mining. You can decide whether you want packages that allow text analytics so that you can start to generate insights from unstructured data, too, or stick to more traditional statistics. Different teams or users can also opt to have different packages, all on the same platform.
The flexibility is not just seen in the range of software applications or volume. The best analytics-as-a-service packages can also integrate open source software, supporting programming in multiple languages, and not just proprietary ones. This is important because many data scientists have preferred programming languages – R or Python, for example – and the company’s choice of analytics platform needs to support this, rather than try to force compliance with an unnecessarily rigid standard.
For those who lack the in-house skills as well as technical computing power, there is another option, known as “results-as-a-service.” This is rather like a consultancy contract, where data is delivered to the service, and results emerge. Instead of hiring the software to do the analysis, you hire the capacity to generate the answers and insights, cutting out a stage in the process. This can be particularly useful as a way of starting to use analytics, perhaps while in-house data scientists are recruited or trained. It can also provide answers to very specific business problems initially to secure executive buy-in, before you scale up and embed more general solutions across the organisation.
Advanced analytics on a shoestring
The flexibility of analytics-as-a-service is the main reason why it is increasingly possible to access analytics, including AI, on a shoestring. It requires no big, upfront investment and is almost infinitely scalable to match demand. For those thinking about taking the plunge, it is an effective way to dip your toe in and test the temperature.
The flexibility of analytics-as-a-service is why it is possible to access #analytics incl #AI on a shoestring. #Banking #CloudAnalytics Click To TweetFind out more about how cloud analytics and results-as-a-service can help your organisation.
For inspiration:
https://www.plugandplaytechcenter.com/resources/banking-machine-learning/
https://www.techemergence.com/machine-learning-in-finance/
https://gomedici.com/how-banks-regulators-are-applying-machine-learning/
https://www.sas.com/en_gbus/services/offerings/results-as-a-service.html
1 Comment
This is a really useful blog. I really enjoyed reading this blog. It was explained and structured with perfection. It helps youngsters to know more about it.