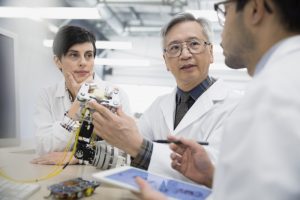
Advanced analytics is an important part of artificial intelligence (AI). Machine learning, or the ability of computers to learn from data, rather than through programming rules, means that more complex problems can be addressed than would otherwise be possible. It is significantly easier to supply lots of data and examples to an algorithm than to program it for every eventuality especially in image recognition and understanding natural language.
Despite the promise of AI, however, many organisations are still struggling to conceptualise the possibilities of machine learning, and to understand what they might be able to do by using these techniques in terms of monitoring and delivery. Far more are also failing to realise the value from their investments, or to do so within a reasonable time frame.
Examples and case studies
One of the best ways to understand and conceptualise what might be possible from using machine learning is to look at some case studies or examples. Two in particular may be helpful:
Using edge computing techniques with high-definition cameras around the pitch generates large amounts of data about each player and the precise location of the ball whenever it is touched. Deep learning techniques allow SciSports to generate ‘heat maps’ for individual players to show, for example, where on the pitch they were when they set up a chance for a goal, or scored. This, in turn, allows analysis of likely success and failure of each action, and to model behaviour for each player. Eventually, the aim is to have real-time scoring for players using the deep learning algorithms, and therefore enable identification of ‘rising stars’ and undervalued players by benchmarking performance against peers.
- VU medisch centrum is exploring medical image recognition on scans
A new project is hoping to use AI and machine learning to explore the idea of using image recognition to assess the likely response of particular tumours to chemotherapy, and predict outcomes. The work will use a huge amount of data from a large-scale clinical trial in the Netherlands on colorectal cancer patients with liver metastases. Data from before and after treatment, including on the DNA of each tumour and from CT scans, will be used to train an algorithm to predict outcomes of chemotherapy, and so help to identify the best course of treatment. The hope is that the algorithm will reach levels of accuracy that are close to or comparable to human experts, as this would save an enormous amount of clinician time and effort, and also increase standardisation of treatment across centres. The most important part of predicting outcomes in cancer research is the fact the environment should secure transparent and monitored as the data is personal.
Realising value
These use cases make it much easier to conceptualise the use of machine learning techniques. It is, however, harder to realise the value in practice. More and more companies and organisations are turning to analytics platforms for this purpose, and particularly as a way to accelerate the value-generation process.
Platforms can — and should — provide support across the whole of the analytics lifecycle for AI applications, from data preparation through to model deployment, with a wide range of AI techniques available, including natural language processing and image recognition. By encouraging collaboration in a single, shared environment, they significantly speed up time to value for getting AI models into production. This makes a huge difference. Other benefits of a good platform include that it is infrastructure-independent: it should, for example, be possible to run it either on-premises or in the cloud, making it extremely flexible, but also ensuring that the host organisation remains in control of costs and usage.
A good platform should also allow easy scaling-up of prototypes, because of the support across the whole analytics lifecycle, and enable the use of open source and vendor-specific software as and when required, through the use of APIs. There will always be specialised systems, and platforms need the ability to integrate them effectively and seamlessly. The ability to absorb and take account of feedback is also essential. These capabilities cover the practical aspects of training, developing and deploying models. This ensures that the process is as rapid and seamless as possible, and therefore that value can be generated quickly.
A good #platform can provide support across the whole of #analytics lifecycle for #AI applications, be infrastructure-independent and allow easy scaling-up of prototypes. #machinelearning Click To TweetGetting the most from an investment
Few organisations have time or resources to waste. Analytical resources are particularly valuable, because data scientists are both scarce and expensive. It therefore makes sense to maximise the use of this resource by ensuring that systems are in place to make the processes they serve as smooth as possible. Analytics platforms are one very good option for this.