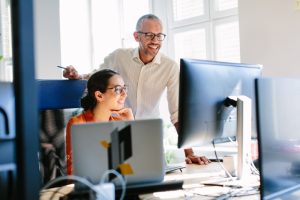
In my previous post, I talked to Prenton Chetty, Head of Analytics at Nedbank, about changes to analytical projects. In this post, we discuss how data science teams are organised and the operational challenges of running this type of team.
Do you think data science teams should be centralised or decentralised?
To be an effective data science team, you need several components: the hardware, the software and the people skills. Each of those is pretty complicated to get right. On balance, I think it’s no use having data scientists sitting by themselves. They need the data, and the community of knowledge from other people, the right software, and so on. So I think that an effective data science team really needs to be centralised.
An effective #DataScience team needs several components that are complicated to get right. It needs to be centralised. #banking Click To TweetWhat would you say are the main challenges on a typical analytical data science project?
Sourcing the data is always a challenge, although we have Management Information teams to help with that, so it is probably not as painful as in many other institutions. The other issue is tying what we do into the business objectives. Sometimes business units understand their problem, but it’s hard for them to articulate it to an analyst, and there is another disconnect when we feed the solution back to the business. For me personally as a manager, that’s an important part of my role, to eliminate the disconnect, and interpret between the two.
If you had to write a paper on best practices, what key process or methodology would you follow?
I think the key thing is to understand the business problem. A lot of people build models that do not specifically solve the business problem. Say the business wants to increase sales. Building a propensity model doesn’t actually do that – it just tells you who is most likely to take up a product. If you look at the actual volume of sales, the model might have no impact. Even though you might have a high hit rate, you may not actually increase sales because those customers would have taken up the product anyway. For me, the critical part is to understand the business problem and ask whether what you’re building is actually going to solve it. The analytics piece is simple by comparison. You need to be able to give business the answers, and help them understand what to do as a result.
Who are your typical stakeholders when you are solving these problems, and how do you work with them?
We generally get everybody involved right at the beginning. Then once we have a plan going forward, everyone has specific responsibilities so we can get the right people in each meeting. But upfront, it’s critical to have everyone around the table because otherwise you may miss a scenario. People with different backgrounds bring different ideas, so you have more chance of picking up issues. Once we’ve done that, we can focus on the specifics and the precise input that we need.
On average, how long does it take to complete a project?
The development time is largely dependent on the business input. If the business unit does not want much input, they just need a model to do X, and will let us get on with it. Then we can build it really quickly, including sourcing the data. However, when we’re working more closely with the business unit to identify variables and work out which ones to use, and where to get the data, that takes a lot longer – months, rather than weeks. And when you introduce a new variable, you also need to consider its stability, accuracy, how quickly it needs updating and so on. It all takes time.
How easy it is for you to take work from a particular project and replicate it to other business areas?
You can definitely take models and replicate them in other areas. We’re also finding that we’re starting to build up experience that applies elsewhere. When someone proposes something, I can immediately say, OK, we’ve tried this before and this where we went wrong, this is what worked. This is really valuable.
You can definitely take models and replicate them in other areas. This is really valuable.
Let’s talk about achievements and failures. What have you learned most from your failures?
I think the biggest lesson is about whether you are really solving the business problem. As analysts, we like to think that we’ll build a model and it will solve the problem, but we have to be honest with ourselves and realise that sometimes things don’t work out the way you think they will. You can build a great model, but three months later, nothing has happened. You have to be willing to accept that you could have a terrific model statistically, but it may not actually have business impact. Then you have to ask yourself what you missed, and why the model is not effective.
You are often tasked with explaining complex things to internal business users. How do you achieve this?
I tend to start at a very high level and give as little information as possible. Whenever I do a presentation, the graphs and outputs are very simple, and it almost looks like we did no work. If the user asks for more information, then we can go into it, but I find that if you try to explain everything, people get a bit lost, so I try to explain only what they need to know. I find that is the most effective way.
This blog post is the 3rd and last part of an interview with Prenton Chetty, Head of Analytics at Nedbank. If you woud like to read the whole interview, you can find the 1st part here: How data science revamps the way we do business and the 2nd part here: Artificial intelligence and changing analytics .
1 Comment
I read this polst completely about the comparison of latest and preceding technologies, it's awesome
article.