The importance of real-time engagement is rapidly growing in companies around the world. The vast majority of customers now want and expect personalised experiences, tailored to fit their preferences and habits. This type of personalisation is only possible, however, with really good real-time analytics to provide insights, and suitable systems to take action as a result. Defining the scope of a suitable analytics platform, however, could be more challenging.
Defining the requirements across three domains
An analytics platform needs capabilities across three areas: data, discovery and deployment. Data is the foundation, the raw material brought to the platform for analysis. Without the right data, no useful insights will be possible, and there are a wide range of types. Discovery covers the exploration of the data, including training models and the use of the platform in different places, including at the source, and where data is stored. Deployment is where the value emerges: applying the models and getting insights, then acting as a result. This phase includes presenting information in reports, and also reintegrating it back into operational systems for automated decision making. The latter is what enables personalisation in a real-time context to digital channels.
As a relatively easy first requirement in the data domain, it is clear that a suitable analytics platform to support real-time engagement will need to be able to integrate data rapidly from a wide range of sources, including devices and machines connected to the Internet of Things (IoT). As far as possible, the platform should be able to draw data from every conceivable source, and also have the flexibility to expand to more sources if necessary. The requirement for speed means, effectively, being able to process and integrate data as it arrives. This can involve analysing data at the source, but also combining multiple sources in real time.
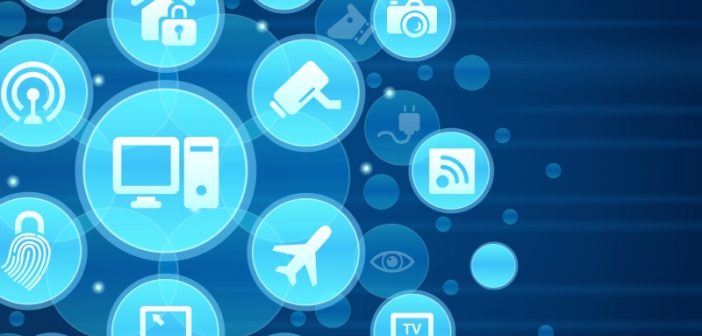
Data is increasingly going from being just structured information to unstructured data sources, such as text, voice and images. And in the future, we will probably see this information used not only to understand questions by using natural language processing, but also, for example, to actually extract additional “soft” information such as emotions and feelings from a combination of images, text and sound. This will of course greatly increase the personalisation. For the time being, however, real conversation and empathy are still out of reach for most analytics platforms.
Deriving insights from more, and growing numbers of, data sources is possible at sensible cost and timeframes. Enterprise-wide analytics is within reach, providing you have a high data management quotient. Test it here.
Future-proofing is important. The only thing we know about the future is that we cannot usually predict what it entails. For that reason, the most important thing is future-proofing. From a platform perspective, this means having a cloud-ready, scalable and adaptable platform. We need to be able to start somewhere with getting a model implemented and actually in use in real-time in our channels, but then continue adding models/additional information iteratively, no matter if it is just adding a new field describing the customer or advanced algorithms for extracting data from text or pictures.
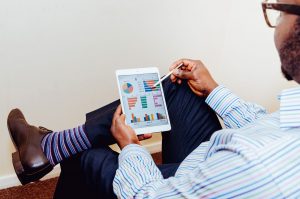
In discovery and deployment, the platform must be able to create, deploy, monitor and retrain models. It must also be able to move seamlessly between discovery and deployment phases, as models are trained, deployed, monitored and retrained. Self-tuning algorithms are possible with good (trustworthy) machine learning capability. This model training and retraining, however, is a challenge to achieve with the drive to reduce data at the edge. Much analytical work can be done at the edge to facilitate faster decisions, but this needs to be balanced against requirements elsewhere. The same platform can, and should, have both capabilities. Even if there is a lot of focus on the advanced real-time aspects, it also needs to be possible to easily generate tailored reports for business users, such as directors, while still keeping the flexibility to allow nontechnical users to tailor reports and visualisations.
Does an analytics platform also require data management capability? I think the answer may well be yes, both for self-service data management and more productionised data management. The self-service parts allow analysts and business users to transform data to quickly verify if the data should be used in models/decisions. This is important both for the efficiency of the analyst/business user and for reducing the amount of data that needs to be put into the more productionised flows, since some of the data can be disregarded based on the work done by the analysts/business users. Having the self-service and production flows in the same platform also reduces the time to deployment since the work done by the analyst/business user can be used as both a prototype and a requirement specification to reduce the actual work of building the production flows for deploying the data management.
This will generate trustworthiness of the platform, as well as position the platform as the most effective way to work with data and analytics. This is essential in ensuring use and uptake.
Implementing an analytics platform has shown great promise in growing the strategic value of analytics and in fostering innovation. These are just two findings from new research consisting of in-depth interviews with professionals in 132 organizations and a global online survey. Download your copy here.
Fixed or tailored?
These requirements are all important in considering an analytics platform for real-time and predictive engagement. It is, however, almost more important to ensure that the platform is fit for the organisation. The best analytics platform in the world will not give value if it is over-engineered for the organisation, or if users cannot manage its interfaces and tools. The precise scope will therefore be determined by the organisational needs.
An #AnalyticsPlatform must be precisely scoped determined by the organisational needs. #Analytics Click To TweetFor example, how easy is it to predict the level of need? And how experienced are the users? If the answer to both these questions is “not very,” then the platform needs to be easy to deploy and scale, or value will not be obtained. A more flexible and adaptable approach, such as analytics-as-a-service, can be helpful in providing both ease of deployment and scaling, and can therefore be particularly helpful for small and medium-size enterprises.
The bottom line, therefore, is that it is relatively easy to set out some of the basic elements of an analytics platform to manage real-time engagement. It is, however, also important to look at the organisational context, to ensure that there is also a good fit with that. And most importantly, even if the vision is to have an enterprisewide analytical platform, start with a concrete use case that your company actually expects will drive value, and use that as the first step.