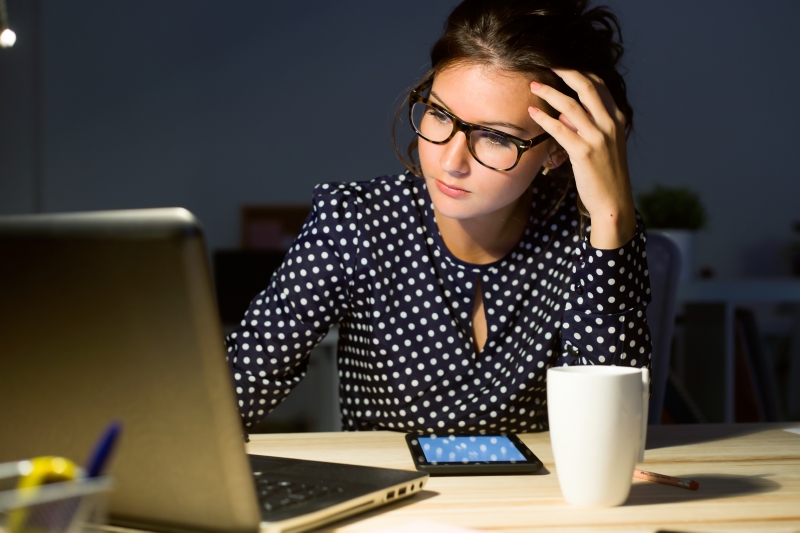
Data integration teams often find themselves in the middle of discussions where the quality of their data outputs are called into question. Without proper governance procedures in place, though, it's hard to address these accusations in a reasonable way. Here's why.