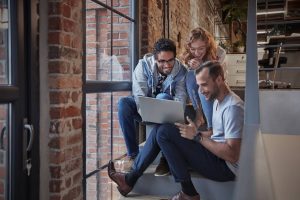
Without a steady supply of relevant, high-quality data that’s ready for analytics, data scientists can’t use analytics to its greatest potential. In the worst cases, organizations that are not equipped to be data-driven will fail to innovate and thrive. How can you ensure that a steady supply of analytics-ready data is available at your organization? Start by understanding the different approaches to preparing data so you can choose the option that’s best for each situation you encounter.
There are three overarching approaches to preparing data for analytics:
- Give users self-service tools for data preparation.
- Use traditional data management technologies, such as extract, transform, load (ETL) and data quality tools.
- Automate the data pipelines using software technologies like replication, streaming, virtualization and machine learning.
So, which approach is best? The bottom line is that all these choices are valid, and each one serves a purpose. In my opinion, the technology you should choose depends on your requirements, the intended business uses, and the needs of the user. Let’s look at each option below. I’ll include a breakdown for each one that shows which users are most likely to benefit from that approach, the time to value you can expect from it, and the overall pros and cons.
Self-service data preparation tools
Self-service tools are ideal for users who want to manage the data themselves. This is an especially good approach if you have limited power users or data experts and you need to reduce reliance on those resources. With self-service data preparation, the user decides how to manage their data. You give them access to the data, and the self-service tools provide the data management they need. Then they get to decide what to do with the prepared data. Here’s a way to evaluate this approach:
- Users: Business analysts, data scientists, data engineers.
- Time to value: Rapid results are typical. Short learning curve.
- Pros:
- Puts end users in control.
- Provides fast and easy access to data.
- Is ideal for one-off requests.
- Is low cost, reduces workload on data management professionals, and gets simple to moderately complex jobs done.
- Cons: Raises the risk of creating more data silos. Provides limited data governance. Increases risks of data inconsistencies because each person uses different calculations, business rules, etc.
Traditional data management technologies
Traditional technologies such as ETL and data quality are useful for ensuring a healthy data supply that can be used for analytics. These tools are designed to handle both simple and complex needs. They allow data professionals to design, test and deploy processes in a way that makes use of the organization’s data fabric, and they adapt to change easily.
Traditional data management offers a more structured approach to managing data. Instead of focusing on managing a data set for a specific analysis or report, traditional data management is suitable for managing data and ensuring consistency across the organization. These technologies do the heavy lifting and create reusable data assets. This improves the productivity of data consumers who are generally focused on analytics, visualization and making business decisions. Evaluate this approach with these things in mind:
- Users: Data providers, ETL developers, data engineers.
- Time to value: Short to medium. Longer initial learning curve due to data complexity and enterprise integration methodologies.
- Pros:
- Puts data providers and data engineers in control of data management processes upstream in the data life cycle.
- Is ideal for using the data infrastructure and orchestrating complex processes.
- Improves productivity of data management professionals.
- Enables data engineers to design, test and deploy any data of any complexity.
- Tends to result in a single version of the truth, across data silos and user groups.
- Provides accurate data and metadata and has change management capabilities.
- Cons: Not designed for end users. Requires having data specialists who know how to get full value from these techniques. Can take longer to deliver results if there are backlogs.
Automated data pipelines
Automated data pipelines are another way to ensure data is managed and delivered reliably to users. Some examples include: replication, streaming, federation/virtualization, data services and machine learning. Once configured, these technologies can make data available to users automatically, without the need for a human to intervene. Consider these aspects of this approach:
- Users: IT, data providers, ETL developers, data engineers and data consumers (as users of the data).
- Time to value: Short to medium. Longer initial learning curve due to data or system complexity, and configuration requirements.
- Pros:
- Enables data providers to automate how data is managed in processes upstream of the data consumer.
- Is ideal for making use of the data infrastructure.
- Improves productivity for both data management professionals and data consumers.
- Enables data engineers to automate data management. For example, an automated process watches streaming data, detects data of interest (for example, an anomaly), captures the data, uses machine learning algorithms to recommend the best actions to take, and sends the data and the recommendations to a user.
- Cons: Requires specialists to configure and set up the integration points.
Which approach should you use?
The answer: It depends.
All three approaches are complimentary, and no single approach is a silver bullet. But when you consider all of these approaches together, and pick what’s best for each situation, you’ll benefit from optimal speed, effectiveness and efficiency.
Consider an example. In practice, most organizations will choose to use traditional ETL for the heavy lifting of data – to orchestrate complex processes while maintaining the most possible control. At the same time, those organizations may use self-service data preparation tools for last mile data processing, and to meet users’ ad hoc needs. So, deciding on an approach entails finding the right balance between choice and control.
The technology you select to deliver your data may also be driven by other factors. You’ll want to consider:
- Cost (such as your budget of time and economic resources).
- Skills (including IT, big data expertise and analytics skills).
- Governance, risk and compliance (how important is it to get the data right?).
- Human involvement versus automation.
- One-off versus routine data delivery.
- Data availability.
- Performance.
In the end, having choices means you can reliably support the diverse data delivery needs of your organization. Consider which of the three approaches you’re using now – and what you may want to do differently after evaluating the nuances of each option.
See how SAS helps you prepare data for analytics – no coding required