One area that often gets overlooked when building out a new data analytics solution is the importance of ensuring accurate and robust data definitions.
This is one of those issues that is difficult to detect because unlike a data quality defect, there are no alarms or reports to indicate a fault; the user simply infers the meaning of the analytics in an incorrect fashion.
Let’s take a car dealership for example. A new IT manager decides to invest in an analytics solution and provides a dashboard to executives. The CEO wants to know how well the business is growing so they observe customer growth which shows a steady increase over time.
The analytics system receives regular feeds of data from over 250 dealerships across the country but many use their own customer management systems. This is where the problems begin.
Some dealers class a customer as someone who has purchased a vehicle. Other dealers class a customer as anyone who takes a vehicle for a test drive. Others class a customer as anyone who has brought their vehicle in for a service.
Some dealers supply multiple customer records for the same vehicle. For example, I noticed that our local dealer had three customer records on file. One record from when I serviced my previous car, one for my wife’s purchase record and one for the servicing of my current car.
Getting an accurate view of how many unique customers an organisation has is therefore extremely difficult when standards, quality and, importantly, definitions of business entities differ across departments, suppliers and partners.
In the last post I talked about the importance of lineage in delivering data analytics and this applies equally to your data definitions. You need to know who created them, when they were created, what systems and physical data items they relate to, what their change history is and whether they accurately reflect current thinking.
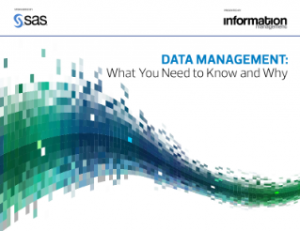
Importantly, there has to be business involvement with the creation of definitions. If you find that during the build of your analytics system all the input around definitions is coming from the IT community then alarm bells need to be sounded. Particularly in older legacy systems, there is a high likelihood that the usage and meaning of a particular data item will have changed and this needs to be reflected in the data definition. Only the business users will know how this change impacts the definition.
A good example of this was a past utilities client that began using a location code of ‘0000’ to denote that a piece of equipment was no longer active and installed. This definition wasn’t fully documented so fixed asset counts were reported inaccurately.
So, the moral of this week’s post is to think carefully about your data definitions when building out your data management strategy for analytics. Whilst poor definitions won’t necessarily cause your reports and dashboards to crash, they may seriously inhibit decision-making ability if actions are incorrectly interpreted.