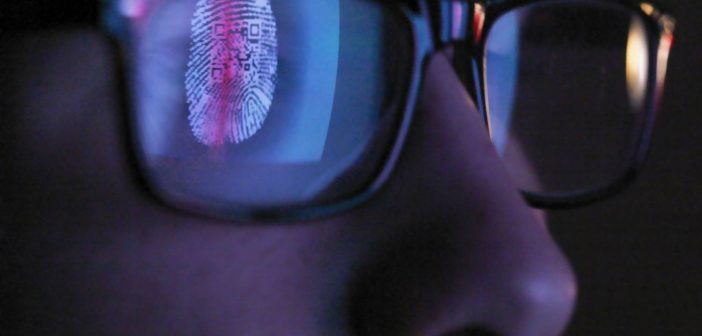
Digital channels open the door to synthetic identity fraud. Luckily, artificial (AI, that is) can defeat synthetic.
Digital channels open the door to synthetic identity fraud. Luckily, artificial (AI, that is) can defeat synthetic.
Helmut Plinke explains why modernizing your data management is essential to supporting your analytics platform.
As the application stack supporting big data has matured, it has demonstrated the feasibility of ingesting, persisting and analyzing potentially massive data sets that originate both within and outside of conventional enterprise boundaries. But what does this mean from a data governance perspective?
In the previous three blogs in this series, we talked about what metadata can be available from source systems, transformation and movement, and operational usage. For this final blog in the series, I want to discuss the analytical usage of metadata. Let’s set up the scenario. Let's imagine I'm a
As I discussed in the first two blogs of this series, metadata is useful in a variety of ways. Its importance starts at the source system, and continues through the data movement and transformation processes and into operations. Operational metadata, in particular, gives us information about the execution and completion
Balance. This is the challenge facing any organisation wishing to exploit their customer data in the digital age. On one side we have the potential for a massive explosion of customer data. We can collect real-time social media data, machine data, behavioural data and of course our traditional master and
Our world is now so awash in data that many organizations have an embarrassment of riches when it comes to available data to support operational, tactical and strategic activities of the enterprise. Such a data-rich environment is highly susceptible to poor-quality data. This is especially true when swimming in data lakes –
Most enterprises employ multiple analytical models in their business intelligence applications and decision-making processes. These analytical models include descriptive analytics that help the organization understand what has happened and what is happening now, predictive analytics that determine the probability of what will happen next, and prescriptive analytics that focus on
As I've previously written, data analytics historically analyzed data after it stopped moving and was stored, often in a data warehouse. But in the era of big data, data needs to be continuously analyzed while it’s still in motion – that is, while it’s streaming. This allows for capturing the real-time value of data
Back before storage became so affordable, cost was the primary factor in determining what data an IT department would store. As George Dyson (author and historian of technology) says, “Big data is what happened when the cost of storing information became less than the cost of making the decision to