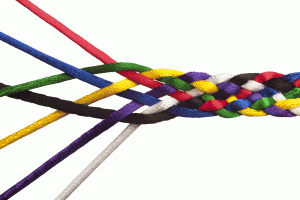
Many of the buzzy applications of AI in healthcare we hear about involve medical IoT, computer vision for radiology or disease prediction. But the fact is, many health institutions that just aren’t there yet with adoption.
With limited budgets and dated systems, can public health agencies tap into the power of AI? Yes! And they don’t need a massive technology infrastructure to start.
There are many promising areas for the application of AI in healthcare and life sciences. However, one area I’ve particularly focused on in the past year has been in risk stratification, especially for those who are often labeled as “high utilizers,” frequently of multiple systems.
Across the country (and world), public and private health systems are recognizing that we need to better coordinate services across health disciplines and even non-health systems, from social services, housing, and even the criminal justice system.
Identifying these high utilizers can be quite challenging, to say the least. Historically, we have often approached this by looking at people with high cost or with many visits or bed days. That’s a place to start but has challenges by typically only looking at siloed data, which doesn’t necessarily help identify people who are cycling through the systems. An individual with a serious illness or accident may be high cost with many bed days, but this is not the type of person targeted by coordination or whole person care programs or initiatives.
Further, when we just look at the highest percentage of utilizers within siloed departments, we may not find those who are receiving duplicative, uncoordinated services across systems. When I was with San Bernardino County, we initially did some “hot spotting” by cross-referencing the top 5% of utilizers from different departments. There wasn’t much overlap, to our surprise. It wasn’t until we went deeper that we saw people who were high utilizers, making an impact on overall county services, but didn’t rise to the highest percentage within a single department. A system-wide view changes our perspective and understanding.

What if there’s a different way to assess risk from a more holistic perspective? What if we brought data (both quantitative and qualitative, utilizing narrative progress notes) together from across departments and systems to find risk factors that might otherwise go unnoticed? Could we develop a risk stratification algorithm tailored to particular geographies, institutions, initiatives, etc.? How might that impact policy? Funding? Inclusion/exclusion criteria? Even provider training? Could this help us gain more upstream insights and interventions?
Please share your thoughts on these questions and on AI in healthcare below! I’d love to hear your approaches to risk identification across systems, as well!
My colleagues and I will be on hand in Orlando at HIMSS February 11-14 to discuss this more. Drop by our booth (4769), or schedule an appointment with me while you’re there!
Then, on February 27, SAS Director of Solution Development, Jay King, and I will be hosting a free webinar on this topic. We’ll go through various ways to use advanced analytics to support whole person care
3 Comments
Hi Josh,
Great article. It makes me think of the basic premise underlying the use of multivariate versus univariate statistics. Why look at only one variable when you can consider multiple. It would seem to me that this is nothing new to analytic systems like SAS, but the means to integrate the variables across multiple data collection platforms is something that is new and more possible than ever before. I think you're absolutely right to say that too often data is siloed and not used in an integrative and insightful way. Thanks for some great insights!
Great points, Loren--thanks for your insights! Indeed, advances in technology have allowed us to tap into far more compute power as well as the ability to leverage different types of data, giving us the ability to gain insights that a human alone would not be able to process. And how can that change policies, funding, and how we help people?!
Pingback: 3 ways data can help homeless youth - Government Data Connection