Over the past year and a half, there has been a subtle shift in media attention from big data analytics to what is referred to as the Internet of Things, or IoT for short. The shift in focus is not intended to diminish the value of big data platforms and analytics, but rather to emphasize its place in the emerging future vision.
The key point has to do with the motivating factors for big data analytics, which we could conveniently differentiate into two categories: operational analytics, and what I might call “strategic” analytics. Operational big data analytics techniques absorb and analyze massive amounts of data to look for immediate benefits within operational applications. These could include overcoming impediments to timely deliveries in the supply chain in real time, or continuously monitoring manufacturing systems to adjust production to market demand. Strategic analytics examines massive amounts of data to identify facets of longer-term opportunities such as refined customer segmentation or retail location placement.
Both of these types of analyses have been done for a long time. However, both of these types of analyses can be made more precise when enhanced with streaming event information. Between the ubiquity of smart mobile devices, the preponderance of sensor-enhanced devices, our increasingly networked environment, we are being pushed to absorb, process, and analyze massive amounts of streamed data in real time. In turn, the increase in the amount of real time data being streamed from a broad spectrum of devices enables the development of more complex analyses and models that are able to leverage event streams.
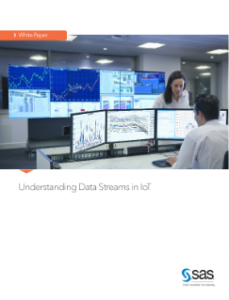
These more complex models blend static analyses associated with the core entities in our business processes (such as customers, products, vendors, trucks, inventories, etc.) with event stream processing engines that not only automate reactions to the events but also encompass analytics to be smart about those reactions. As an example, a simple retail store inventory system might monitor real-time point-of-sale transactions and check each transaction event to see if the shelf stock is close to being depleted and generate an order when stock gets low. A more complex system might combine all retail site point-of-sale data across the network (remember the “Internet of Things”? this is an example!) and not only attempt to preemptively order items in anticipation of low stocks, but monitor the acceleration or deceleration of demand by region in real time to adjust facility manufacturing and shipments as well as adjust prices in real time.
This is just one example of holistic analysis that relies on event stream processing and analytics. These type of scenarios in which processing of different event streams can be integrated with other types of analyses are becoming more common, and this only highlights the importance of event stream processing for enabling predictive and prescriptive analytics.